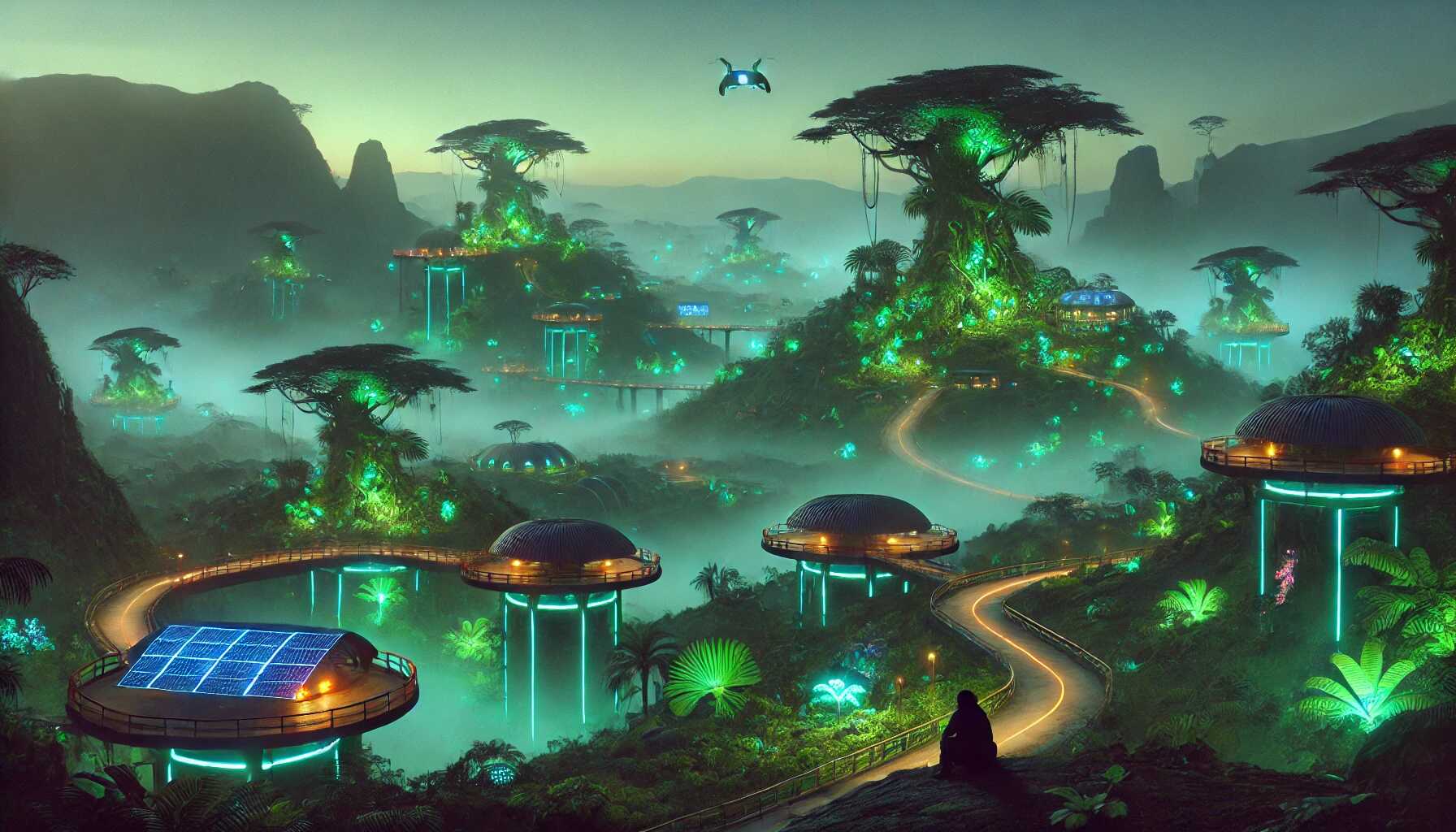
Author(s): Laurin Heilmeyer

TL;DR: Is denormalisation a smart way to improve performance or just a crazy trend? Experts have differing opinions on the benefits and drawbacks of this data optimisation technique. While some argue it can boost performance, others worry about sacrificing data quality. Ultimately, the approach you take depends on your priorities and goals.”
Disclaimer: This post has been created automatically using generative AI. Including DALL-E, Gemini, OpenAI and others. Please take its contents with a grain of salt. For feedback on how we can improve, please email us
Denormalisation: Thoughtful Optimisation or Irrational Avant-Garde?
In the world of data management, denormalisation is a term that often sparks debate and controversy. Some view it as a thoughtful and necessary optimization technique, while others see it as an irrational and avant-garde approach. But what exactly is denormalisation and why does it elicit such strong reactions from data professionals?
To put it simply, denormalisation is the process of restructuring a database by combining multiple tables into one, in order to improve performance. This technique is often used in data warehouses or data marts where speed and efficiency are crucial. By reducing the number of tables and joins, denormalisation can significantly improve query response time and overall system performance.
Perspective on Performance Optimisation
Performance optimisation is a crucial aspect of data management, especially in today’s fast-paced and data-driven world. The ability to quickly and efficiently access and analyze data can make all the difference in gaining a competitive edge. This is where denormalisation comes into play, as it is one of the many techniques used to optimize performance.
However, it’s important to note that denormalisation is not the only solution for performance issues. It should be used in conjunction with other techniques, such as indexing and query optimization, to achieve the best results. It’s also important to carefully consider the trade-offs of denormalisation, as it can lead to data redundancy and potential data integrity issues.
Data Quality and Denormalisation
One of the main concerns with denormalisation is its impact on data quality. As mentioned earlier, denormalisation can lead to data redundancy, which can result in inconsistencies and errors. This is why it’s crucial to carefully plan and implement denormalisation, taking into account the potential impact on data integrity and quality.
Furthermore, denormalisation can also make data management more complex and difficult to maintain. With fewer tables and more data duplication, it becomes harder to track and update data, leading to potential data discrepancies. This is why it’s essential to have a solid data governance strategy in place when using denormalisation.
The Middle Ground: A Balanced Approach
In the end, the debate between denormalisation as a thoughtful optimization technique or an irrational avant-garde approach is not a black and white issue. Like most things in data management, it’s all about finding a balance. Denormalisation can be a powerful tool for improving performance, but it should be used thoughtfully and in conjunction with other techniques. It ultimately depends on the specific needs and goals of the organization. However, no matter the approach taken, performance optimisation and data quality should always be a priority for any data-driven business or project. Balancing these two factors is crucial for achieving successful and efficient data management. Overall, a perspective that prioritises both performance optimisation and data quality is essential for making informed and effective decisions when it comes to denormalisation and data management.
Crafted using generative AI from insights found on Towards Data Science.
Join us on this incredible generative AI journey and be a part of the revolution. Stay tuned for updates and insights on generative AI by following us on X or LinkedIn.