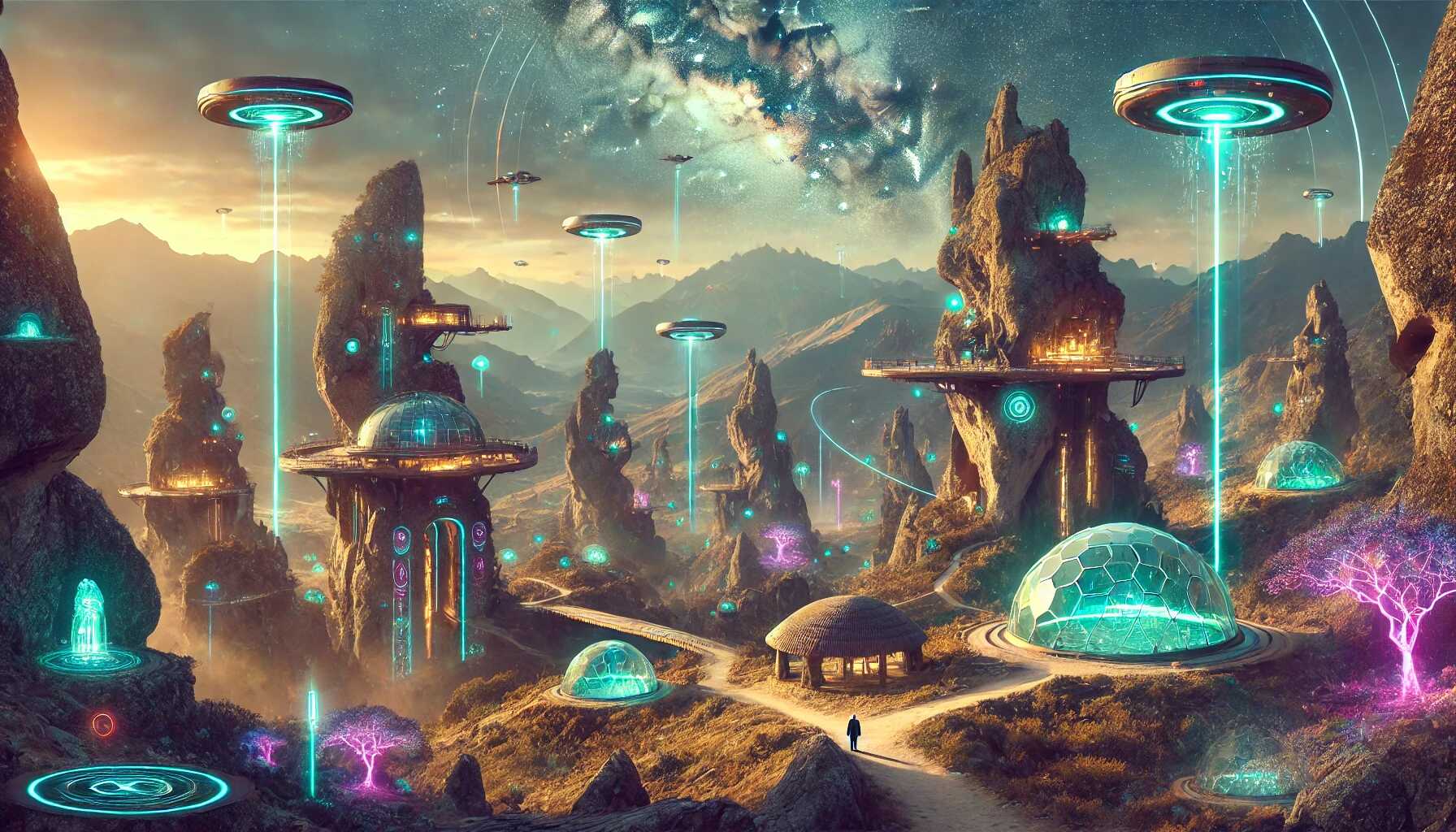
Author(s): Piero Paialunga

TL:DR: Regression analysis is a powerful statistical tool that is used to understand the relationship between a dependent variable and one or more independent variables. It is commonly used in various fields, including economics, finance, and social sciences. However, with so many different types of regression techniques available, it can be overwhelming to determine which one is the most suitable for your specific dataset. In this blog post, we will explore a taxonomy of regression techniques and help you understand which one you should use for your data analysis.
Disclaimer: This post has been created automatically using generative AI. Including DALL-E, Gemini, OpenAI and others. Please take its contents with a grain of salt. For feedback on how we can improve, please email us
Understanding Regression Techniques
Before we dive into the taxonomy of regression techniques, let’s briefly review what regression analysis is. In simple terms, regression analysis is a statistical method that helps us understand how the value of a dependent variable changes when one or more independent variables are varied. The goal of regression analysis is to find the best-fit line or curve that represents the relationship between the variables. This line or curve can then be used to make predictions about the dependent variable based on the values of the independent variables.
Linear Regression
Linear regression is perhaps the most well-known and commonly used regression technique. It is used when the relationship between the dependent variable and the independent variable(s) is linear, meaning that the change in the dependent variable is directly proportional to the change in the independent variable(s). Linear regression is simple, easy to interpret, and can handle both continuous and categorical independent variables.
Multiple Regression
Multiple regression is an extension of linear regression that allows for more than one independent variable. It is used when there are multiple independent variables that may affect the dependent variable. In this technique, the relationship between the dependent variable and the independent variables is still linear, but the model becomes more complex. Multiple regression is useful for understanding the relative importance of each independent variable in predicting the dependent variable.
Logistic Regression
Logistic regression is a type of regression used when the dependent variable is binary or categorical. It is used to model the probability of an event occurring based on one or more independent variables. Logistic regression is commonly used in marketing, medicine, and social sciences. It is a powerful tool for predicting the likelihood of a specific outcome.
Decision Tree Regression
Decision tree regression is a non-parametric technique that uses a tree-like structure to model the relationship between the dependent variable and the independent variables. It is used when the data is non-linear or when there are multiple interactions between the independent variables. Decision tree regression is easy to interpret and can handle both continuous and categorical variables.
Deciding on the appropriate regression technique for a given dataset can be a daunting task, but it is crucial for obtaining accurate and meaningful results. To determine the best approach, a thorough understanding of the data and its characteristics is necessary. This includes considering the type of data, the relationship between variables, and the desired outcome. By carefully evaluating these factors, one can choose the most suitable regression technique for their specific dataset. This taxonomy provides a helpful guide for selecting the best regression technique, but it is important to also consider any unique features or challenges present in the data. Ultimately, the chosen regression technique should be based on a comprehensive analysis and understanding of the dataset to ensure the most accurate and informative results.
Crafted using generative AI from insights found on Towards Data Science.
Join us on this incredible generative AI journey and be a part of the revolution. Stay tuned for updates and insights on generative AI by following us on X or LinkedIn.