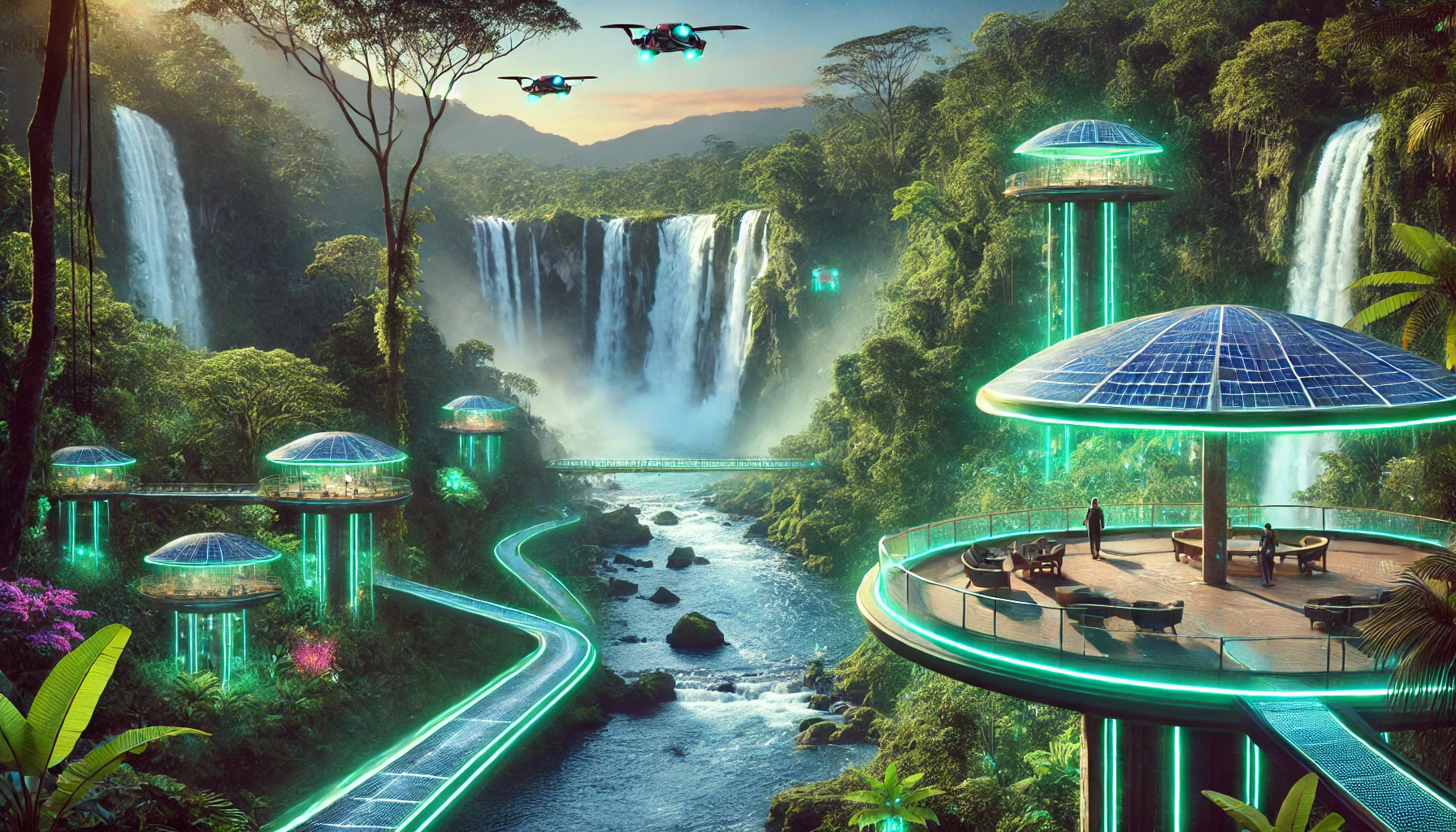
Author(s): Elahe Aghapour & Salar Rahili

TL;DR: New technique for combining specialized language models without the need for large amounts of data. Results show improved performance compared to fine-tuning.
Disclaimer: This post has been created automatically using generative AI. Including DALL-E, Gemini, OpenAI and others. Please take its contents with a grain of salt. For feedback on how we can improve, please email us
Introduction to Specialized LLMs
Specialized LLMs, or Master of Laws programs, have become increasingly popular in recent years as more and more legal professionals seek to deepen their knowledge in a specific area of law. These programs offer focused coursework and hands-on experience in a particular field, such as international law, intellectual property, or tax law. However, as the number of specialized LLMs continues to grow, there is a growing concern about the data burden and potential overlap between these programs.
The Data Burden of Specialized LLMs
One of the main challenges with specialized LLMs is the data burden they create for students, faculty, and administrators. Each program requires its own set of data and information, such as application materials, course schedules, and grading systems. This can be overwhelming for students who are already juggling coursework and other responsibilities. It can also be a burden for faculty who have to manage and track data for multiple specialized LLMs, and for administrators who have to ensure compliance and accuracy of the data.
The Need for Streamlining and Integration
To address this data burden and streamline the specialized LLM process, there has been a push towards merging or integrating these programs. By combining similar LLMs, schools can reduce the data burden and create a more efficient and cohesive experience for students. This also allows for better collaboration and cross-pollination of ideas between different fields of law. However, merging specialized LLMs is not without its challenges.
Challenges and Solutions for Merging Specialized LLMs
One of the main challenges with merging specialized LLMs is ensuring that the unique aspects and strengths of each program are not lost in the process. This can be addressed by involving faculty and students in the decision-making process and finding ways to incorporate the specialized focus into the merged program. Another challenge is managing the logistics and data integration between the different programs. This can be overcome by using technology and software solutions that allow for seamless data sharing and management.
Benefits of Merging Specialized LLMs
Despite the challenges, there are many benefits to merging specialized LLMs. By streamlining the process, students can have a more focused and efficient learning experience. This can also lead to cost savings for both the students and the schools. Merging specialized LLMs can also foster a more collaborative and interdisciplinary approach to legal education, which can better prepare students for the complex and globalized legal landscape.
Conclusion
In conclusion, the merging of specialized LLMs without the burden of excessive data offers a promising opportunity to enhance the effectiveness and efficiency of legal education. By streamlining and integrating various legal fields, this approach has the potential to provide a more comprehensive and practical learning experience for future legal professionals. This could ultimately lead to a better understanding and application of the law in the real world, benefiting both individuals and society as a whole.
Crafted using generative AI from insights found on Towards Data Science.
Join us on this incredible generative AI journey and be a part of the revolution. Stay tuned for updates and insights on generative AI by following us on X or LinkedIn.