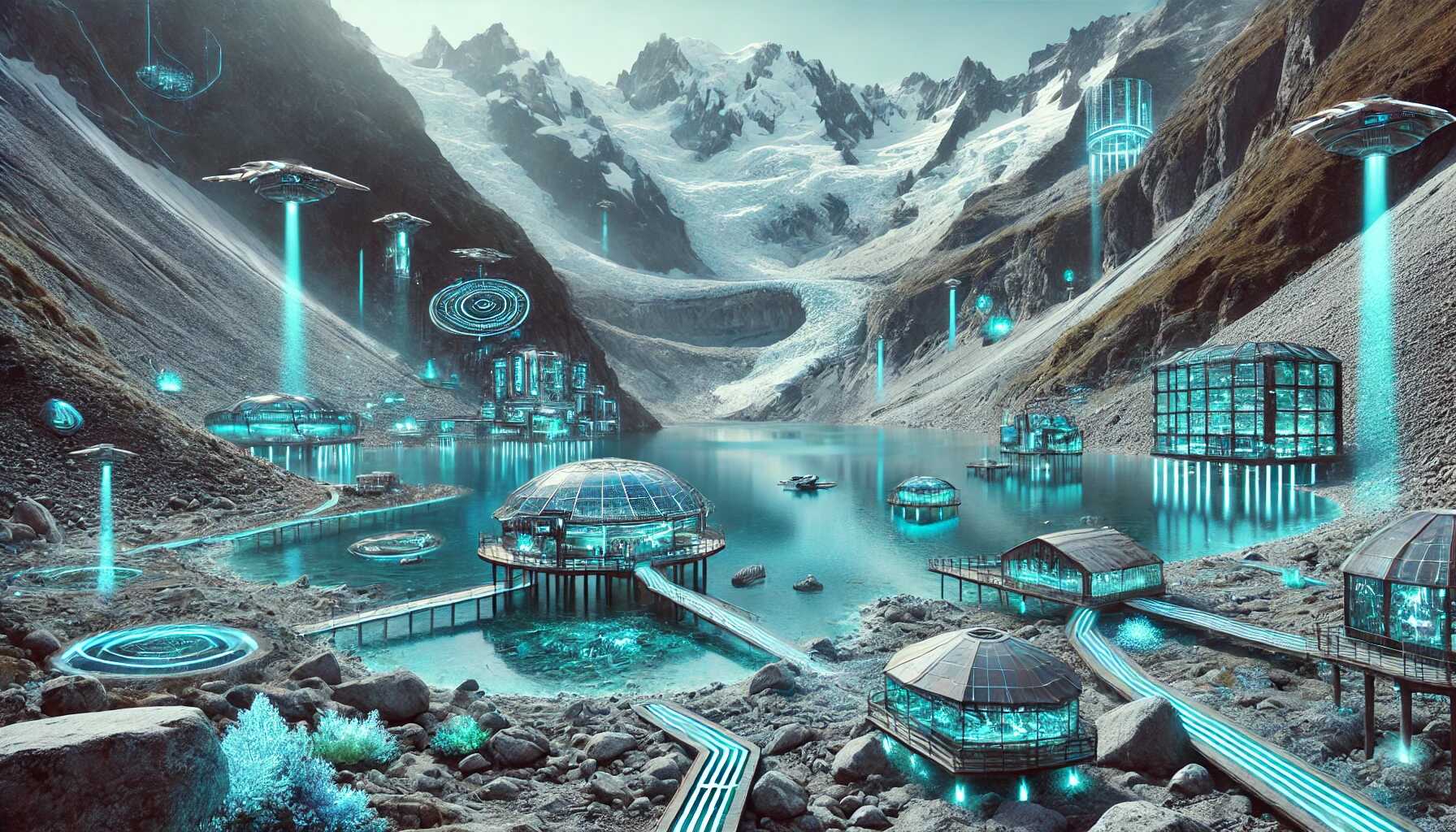
Author(s): Sascha Kirch

Mamba State Space Models are a powerful tool for analyzing images, videos, and time series data. Part 1 of this series introduces the basics of these models and how they can be applied to different types of data.
Disclaimer: This post has been created automatically using generative AI. Including DALL-E, Gemini, OpenAI and others. Please take its contents with a grain of salt. For feedback on how we can improve, please email us
Introduction
State space models have been widely used in various fields such as economics, engineering, and biology. These models are powerful tools for analyzing and predicting time series data. However, their application to image and video data has been limited. In recent years, there has been a growing interest in developing state space models for images, videos, and time series data. In this two-part blog post, we will explore the concept of Mamba state space models and their potential applications in these fields.
What are Mamba State Space Models?
Mamba state space models are a type of state space model that utilizes a Markov chain Monte Carlo (MCMC) algorithm to estimate the parameters of the model. This approach is particularly useful for complex data such as images, videos, and time series, where traditional methods may not be sufficient. MCMC algorithms are able to handle high-dimensional data and can provide more accurate and reliable estimates compared to other methods.
Applications in Image Analysis
One of the main advantages of Mamba state space models is their ability to handle image data. Traditional methods for image analysis often rely on assumptions about the underlying distribution of the data, which may not hold true in real-world scenarios. Mamba state space models, on the other hand, do not require any distributional assumptions and can handle complex and high-dimensional image data. This makes them a powerful tool for tasks such as image segmentation, object detection, and image reconstruction.
Utilizing Mamba State Space Models for Video Data
In addition to images, Mamba state space models can also be applied to video data. Video data is essentially a sequence of images, and therefore, the same principles of Mamba state space models can be applied. These models can be used for tasks such as video tracking, motion analysis, and video compression. By incorporating temporal information, Mamba state space models can provide more accurate and robust results compared to traditional methods.
Predicting Time Series Data
Time series data is another area where Mamba state space models can be beneficial. Time series data is characterized by its sequential nature and often exhibits complex patterns and dependencies. Traditional time series models may struggle to capture these patterns, leading to inaccurate predictions. Mamba state space models, on the other hand, can incorporate both the temporal and spatial dependencies in the data, resulting in more accurate and reliable predictions.
Conclusion
In conclusion, Mamba State Space Models have shown great potential in analyzing images, videos, and time series data. Through the implementation of Part 1, we have seen how these models can accurately capture the complex relationships and patterns present in these types of data. With further development and refinement, these models have the potential to greatly enhance our understanding and analysis of these diverse forms of data.
Crafted using generative AI from insights found on Towards Data Science.
Join us on this incredible generative AI journey and be a part of the revolution. Stay tuned for updates and insights on generative AI by following us on X or LinkedIn.