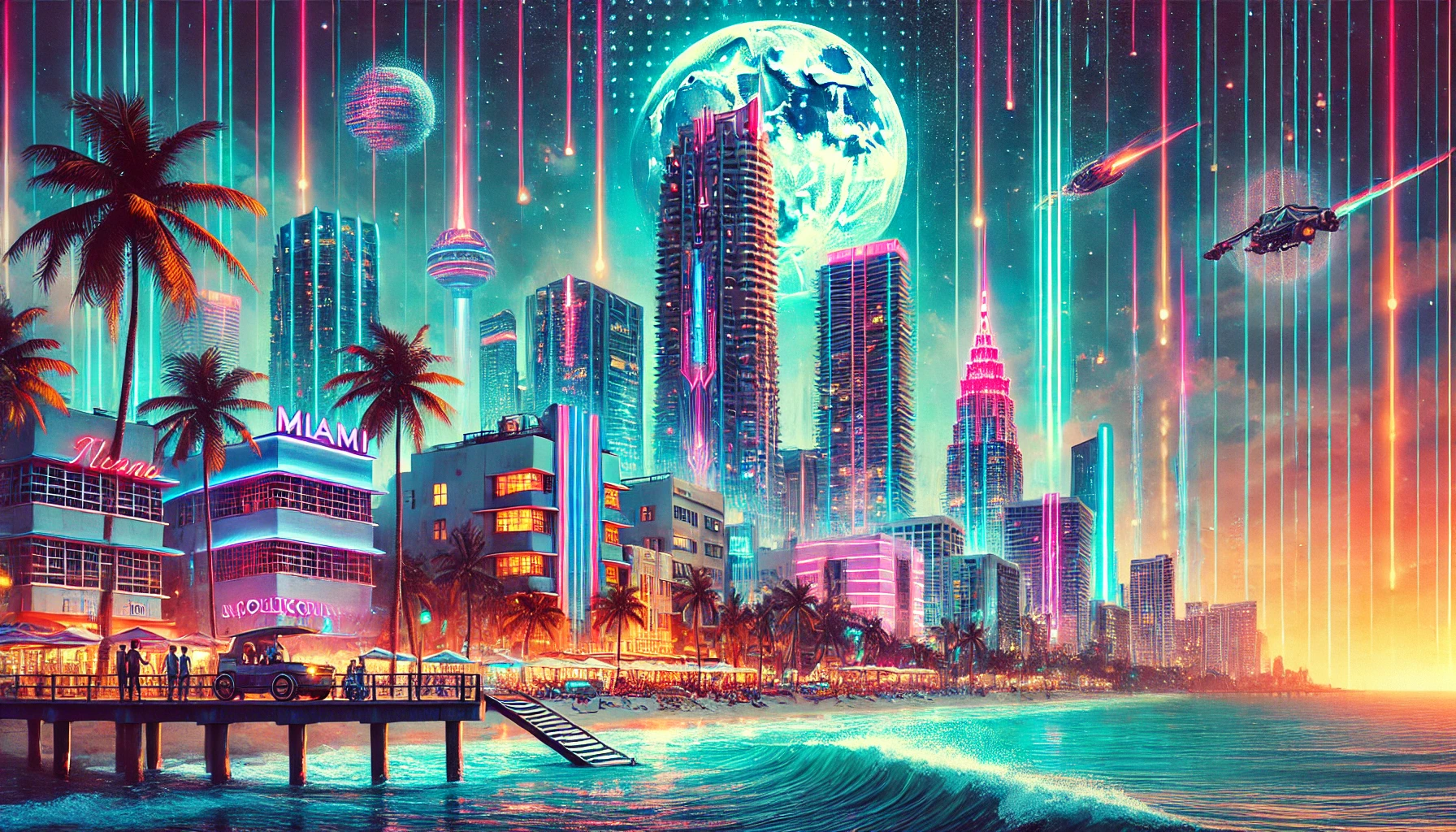

TL;DR: This article explains how stochastic regularization can improve entity embeddings, and explores how neural networks process categoricals and their hierarchies. It provides visualizations to help understand these concepts.
Disclaimer: This post has been created automatically using generative AI. Including DALL-E, Gemini, OpenAI and others. Please take its contents with a grain of salt. For feedback on how we can improve, please email us
Introduction
Neural networks have revolutionized the field of machine learning, allowing for complex tasks such as image recognition and natural language processing to be performed with impressive accuracy. However, understanding how these networks make decisions and perceive data can be a challenge. In this blog post, we will explore the concept of stochastic regularization for entity embeddings and how neural networks perceive categoricals and their hierarchies.
What are Stochastic Regularization and Entity Embeddings?
Stochastic regularization is a technique used in machine learning to prevent overfitting, which occurs when a model becomes too specific to the training data and does not generalize well to new data. This technique involves randomly dropping out some neurons during the training process, forcing the remaining neurons to learn more robust features. Entity embeddings, on the other hand, are a way to represent categorical data in a continuous vector space. This allows for categorical data to be easily incorporated into neural networks, which typically work with numerical data.
Visualizing Stochastic Regularization for Entity Embeddings
To better understand the concept of stochastic regularization for entity embeddings, let’s consider an example. Imagine we have a dataset of customer reviews for a product, with the categories of “positive” or “negative” sentiment. In traditional machine learning, we would represent this categorical data as binary variables, with 1 indicating positive sentiment and 0 indicating negative sentiment. However, with entity embeddings, we can represent these categories as continuous vectors, allowing for more nuanced representations of sentiment. Stochastic regularization helps to prevent overfitting in this scenario by randomly dropping out some of the neurons responsible for learning these embeddings, forcing the remaining neurons to learn more generalizable features.
A Glimpse into How Neural Networks Perceive Categoricals and Their Hierarchies
Neural networks perceive data in a hierarchical manner, with each layer of the network learning more complex and abstract features. When it comes to categorical data, this hierarchical perception can be seen in how the network learns to represent different categories. For example, in the sentiment analysis example mentioned earlier, the first layer of the network may learn to distinguish between positive and negative sentiment, while the next layer may learn to differentiate between different types of positive or negative sentiment (e.g. extremely positive vs. slightly positive). This hierarchical representation allows for more nuanced and accurate predictions.
Conclusion
In conclusion, stochastic regularization for entity embeddings is a powerful technique that helps prevent overfitting in neural networks. By representing categorical data in a continuous vector space, neural networks can better incorporate this type of data into their decision-making process
Discover the full story originally published on Towards Data Science.
Join us on this incredible generative AI journey and be a part of the revolution. Stay tuned for updates and insights on generative AI by following us on X or LinkedIn.