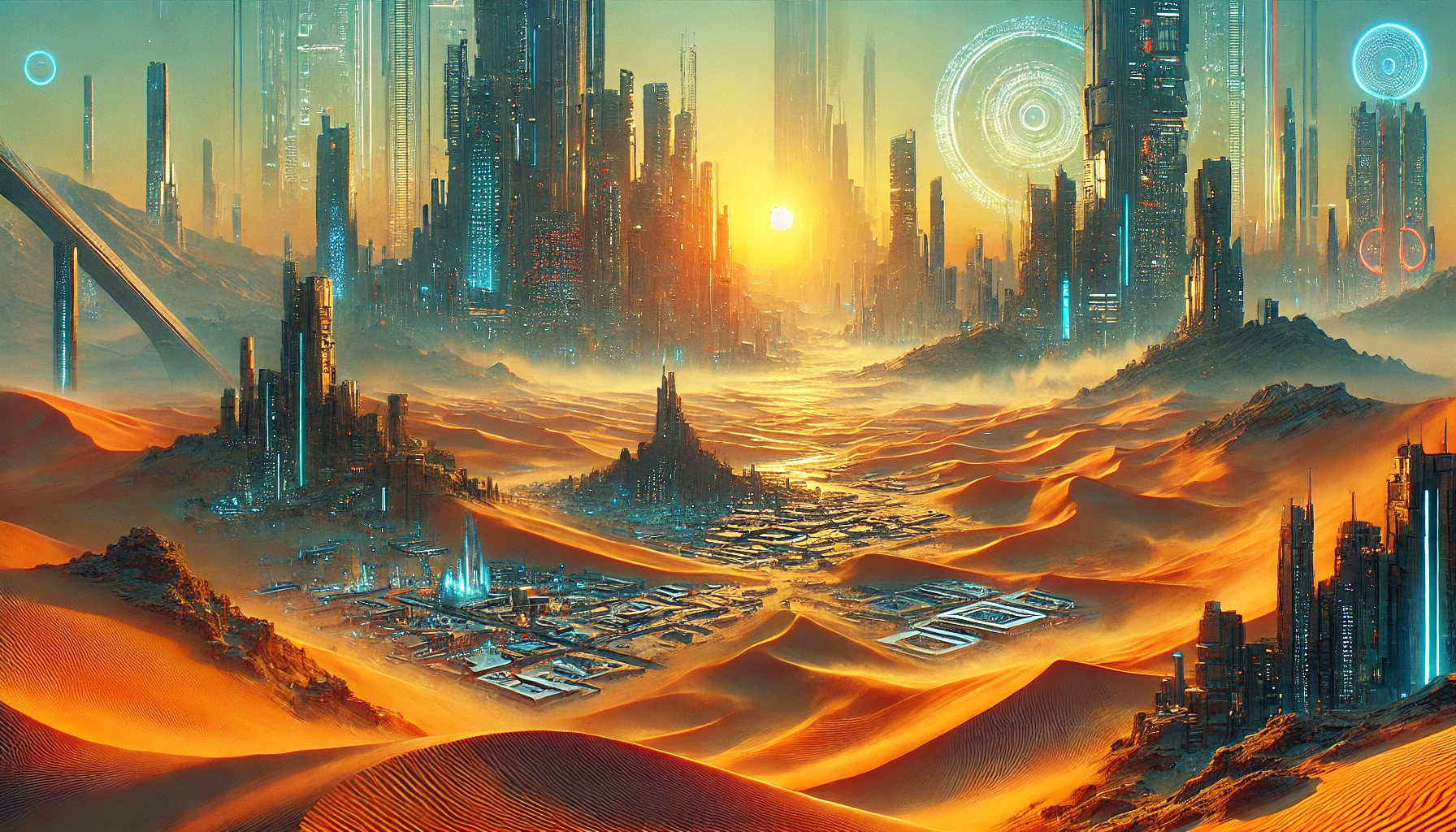
Tl;DR: Data engineering tackles the top 10 data lifecycle problems by providing clear strategies for solving key pain points. These include data quality issues, siloed data, and lack of automation. By implementing effective solutions, data engineering ensures reliable and efficient data management.
Disclaimer: This post has been created automatically using generative AI. Including DALL-E, and OpenAI. Please take its contents with a grain of salt. For feedback on how we can improve, please email us
Introduction:
Data engineering is a crucial aspect of any data-driven organization. It involves the process of collecting, storing, and processing data to make it accessible and usable for analysis. However, data engineering is not without its challenges. In this blog post, we will discuss the top 10 data lifecycle problems that data engineering solves and provide clear strategies for addressing these key pain points.
Problem 1: Data Ingestion
One of the biggest challenges in data engineering is ingesting data from various sources. This can include structured and unstructured data from databases, APIs, and streaming platforms. Data engineers need to ensure that the data is collected accurately and efficiently, without any loss of information. To address this pain point, organizations can implement data integration tools that can handle different data formats and automate the ingestion process.
Problem 2: Data Quality
Data quality is crucial for accurate and reliable analysis. However, data engineers often face challenges in ensuring the quality of the data they work with. This can be due to errors in data collection, duplication, or missing values. To address this issue, organizations can implement data cleansing and validation techniques, such as data profiling and data quality rules, to identify and fix any issues with the data.
Problem 3: Data Storage
As the volume of data continues to grow, organizations face challenges in storing and managing it effectively. Traditional storage solutions may not be able to handle the massive amounts of data generated by businesses today. To address this problem, organizations can adopt cloud-based data storage solutions that offer scalability and cost-effectiveness. They can also implement data partitioning and compression techniques to optimize storage space.
Problem 4: Data Processing
Data processing is a critical aspect of data engineering, as it involves transforming raw data into a usable format for analysis. However, data engineers often face challenges in processing large volumes of data in a timely and efficient manner. To address this pain point, organizations can leverage distributed computing frameworks, such as Hadoop and Spark, to parallelize data processing and improve performance.
Problem 5: Data Governance
Data governance refers to the overall management of data within an organization. It involves defining policies, procedures, and standards for data collection, storage, and usage. Data engineers play a crucial role in ensuring that data governance is implemented effectively. To address this pain point, organizations can establish a data governance framework and assign roles and responsibilities to different teams to ensure data is managed in a consistent and compliant manner.
In conclusion, data engineering plays a crucial role in solving the top 10 data lifecycle problems. By implementing clear strategies, data engineers can effectively address key pain points such as data quality, integration, and governance. By addressing these challenges, organizations can ensure the accuracy, reliability, and efficiency of their data processes, ultimately driving better decision-making and business success.
Discover the full story originally published on Towards Data Science.
Join us on this incredible generative AI journey and be a part of the revolution. Stay tuned for updates and insights on generative AI by following us on X or LinkedIn.