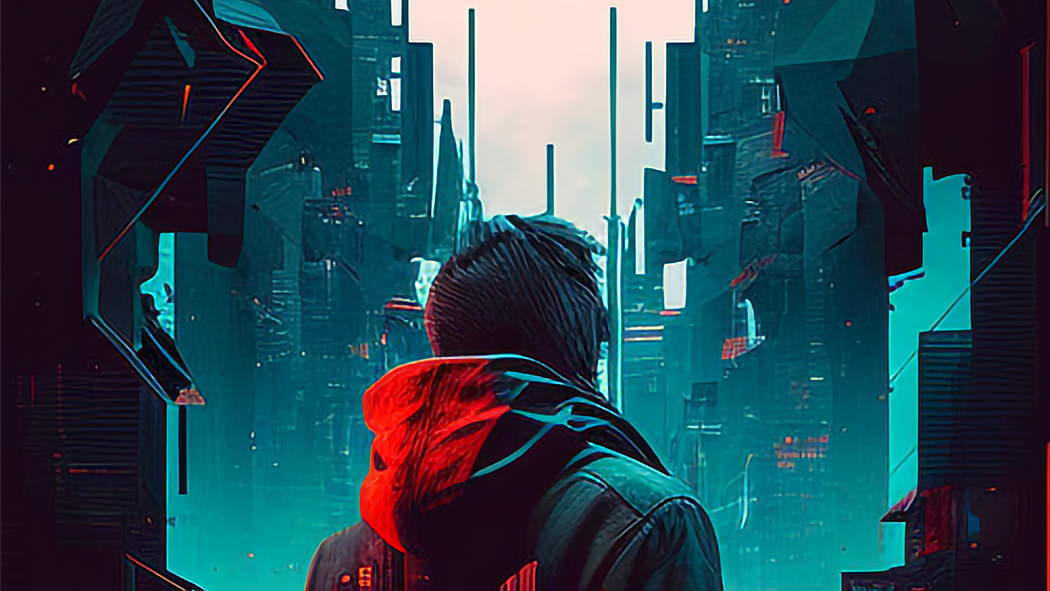
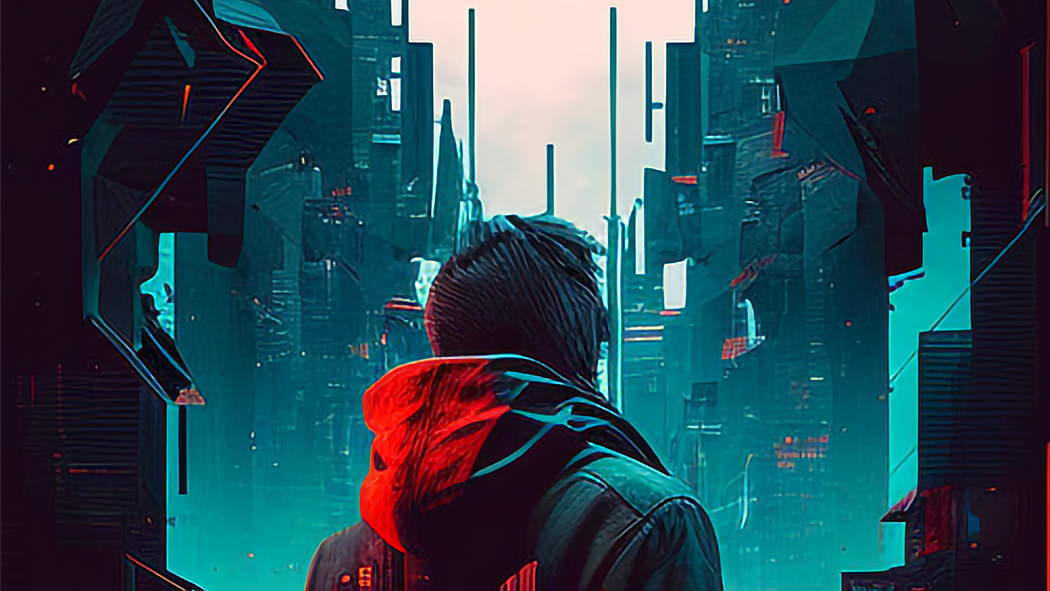
TL;DR:
Hallucinations in generative AI refer to instances where AI generates content that is not based on input data, leading to potentially harmful or misleading outcomes. Causes of hallucinations include over-reliance on patterns, lack of diverse data, and the complexity of large language models. To prevent hallucinations, we can use diverse data, input monitoring, explainability, quality assurance, and human oversight. Ensuring the responsible and ethical use of generative AI requires transparency, explainability, and taking the necessary precautions.
Disclaimer: This post has been created automatically using generative AI. Including DALL-E, and OpenAI. Please take its contents with a grain of salt. For feedback on how we can improve, please email us
Generative AI has been making waves in the tech industry for its ability to generate text, images, and even videos that seem almost indistinguishable from those created by humans. This has led to numerous breakthroughs in various fields, from language translation to video game development. However, with the power of generative AI comes a significant risk: the potential for hallucinations.
What Are Hallucinations in Generative AI?
In generative AI, hallucinations refer to instances where the AI generates content that is not based on any input data. This can occur when a machine learning model generates something that is not present in the training data or when the model relies too heavily on biases or patterns that it has learned from the data. In some cases, the model may generate content that is entirely false, leading to potentially damaging consequences.
The issue of hallucinations is not new. In fact, it is a well-known problem in the field of AI. However, with the growing prevalence of generative AI, the potential for hallucinations has become a more significant concern.
What Causes Hallucinations in Generative AI?
There are many potential causes of hallucinations in generative AI. One common cause is the over-reliance on patterns and biases that the model has learned from the data. For example, if a language model has been trained on data that includes a specific type of language or syntax, it may generate content that is heavily influenced by those patterns.
Another cause of hallucinations is the lack of diverse data. If a generative AI model has only been trained on a small set of data, it may generate content that is not representative of the larger population. This can lead to biases and inaccuracies in the generated content.
Finally, some hallucinations may be caused by the generative AI model itself. Large language models, such as GPT-3, are incredibly complex, and it can be challenging to understand exactly how they generate content. In some cases, the AI may generate content that is not based on any specific input data, leading to potentially damaging consequences.
The Implications of Hallucinations in Generative AI
The potential for hallucinations in generative AI has significant implications, particularly in areas such as finance, healthcare, and law. For example, a language model that generates false information about a stock could lead to significant financial losses for investors. In healthcare, a model that generates false diagnoses or treatment recommendations could have life-threatening consequences. And in the legal field, a model that generates false evidence could lead to wrongful convictions or acquittals.
Moreover, the ethical implications of hallucinations in generative AI are significant. It raises questions about the responsibility of developers to ensure that their models do not generate harmful or misleading content. It also highlights the need for transparency and accountability in the development and use of AI.
How to Address Hallucinations in Generative AI
The potential for hallucinations in generative AI is a significant concern, but it is not insurmountable. There are several ways in which this issue can be addressed:
- Diverse Data: One of the most important steps in addressing hallucinations is to ensure that the generative AI model is trained on diverse data. This can help prevent the model from relying too heavily on patterns and biases.
- Input Monitoring: Another approach is to closely monitor the input data that is fed into the model. By ensuring that the model is only generating content based on valid input data, the risk of hallucinations can be significantly reduced.
- Explainability: Large language models like GPT-3 are incredibly complex, making it challenging to understand how they generate content. Developing techniques for understanding how the model generates content can help reduce the potential for hallucinations.
- Quality Assurance: Before deploying a generative AI model, it is crucial to perform quality assurance testing. This can help identify potential issues, including the risk of hallucinations.
- Human Oversight: Finally, having human oversight in the generative AI process can help prevent the potential for hallucinations. By having humans review and approve the content generated by the model, the risk of false or misleading content can be significantly reduced.
Final Thoughts
Generative AI has incredible potential, but it also comes with significant risks. The potential for hallucinations in large language models is a concern that must be addressed to ensure the ethical and responsible use of AI. By taking steps to address the potential for hallucinations, we can unlock the full potential of generative AI while minimizing the risks.
Machine learning engineers have a responsibility to design and build AI models that are transparent, explainable, and ethical. By taking the necessary precautions, we can ensure that generative AI continues to make a positive impact on society while minimizing the risks.
Join me on this incredible generative AI journey and be a part of the revolution. Stay tuned for updates and insights on generative AI by following me on Twitter, Linkedin or my website. Your support is truly appreciated!
Book recommendations
Building LLM Powered Applications