TL;DR:
This blog post explores the process of generating futuristic yet realistic scenery images of Peru using AI tools. We discuss the inspirations behind each prompt, the challenges faced in creating these images, and the insights gained from the experience. The post delves into the details of ten images, showcasing the blend of Peru’s natural beauty with sci-fi elements to create unique visual interpretations and the insights gained from the process.
In recent years, generative AI has opened up new possibilities for creating stunning visual content, offering a blend of creativity and technology that is changing how we perceive art and design. In this post, we dive into the fascinating world of AI image generation, focusing on a series of futuristic yet realistic images of Peru, created using AI models. These images were crafted with a focus on integrating the natural beauty of Peru’s landscapes with futuristic, sci-fi elements.
Disclaimer: This post has been created with the help of generative AI. Including DALL-E, Gemini, OpenAI and others. Please take its contents with a grain of salt. For feedback on how we can improve, please email us
Harnessing AI for Image Creation
Generative AI has revolutionized visual content creation by combining advanced algorithms with artistic creativity. This post explores AI-driven techniques for creating images of Peru that merge its natural beauty with futuristic themes. The focus is on utilizing models like DALL-E to generate visuals that incorporate both historical and sci-fi elements.
The Role of Prompts in AI Image Generation
Prompts serve as the blueprint for AI models, guiding the creation of complex images. In our project, carefully crafted prompts were used to integrate Peru’s landscapes with futuristic elements. For example, Machu Picchu’s prompt included instructions for blending ancient ruins with advanced architectural designs, utilizing a color palette that combines natural and neon hues.
Overcoming Challenges in Image Synthesis
Balancing the realism of Peru’s landscapes with futuristic aspects posed significant challenges. AI models needed precise tuning to maintain the essence of locations like Rainbow Mountain while introducing neon accents. The task required iterative adjustments to ensure a seamless fusion of traditional and innovative elements.
Key Insights from the Image Generation Process
The project highlighted AI’s capability to interpret abstract concepts and apply them to specific landscapes. For instance, the AI-generated images of the Amazon Rainforest demonstrated a successful blend of natural foliage with high-tech structures. This showcases AI’s potential in envisioning sustainable futures through imaginative visual narratives.
Showcasing Ten Futuristic Images of Peru
Here are ten images that exemplify the fusion of Peru’s landscapes with futuristic themes using image generation:
Machu Picchu
Machu Picchu is located in the Cusco Region of Peru, specifically in the Urubamba Province. It is situated on a mountain ridge above the Sacred Valley, approximately 80 kilometers (50 miles) northwest of the city of Cusco. The site is perched at an altitude of about 2,430 meters (7,970 feet) above sea level and is surrounded by lush cloud forests and towering peaks, making it one of the most iconic and visited archaeological sites in the world.
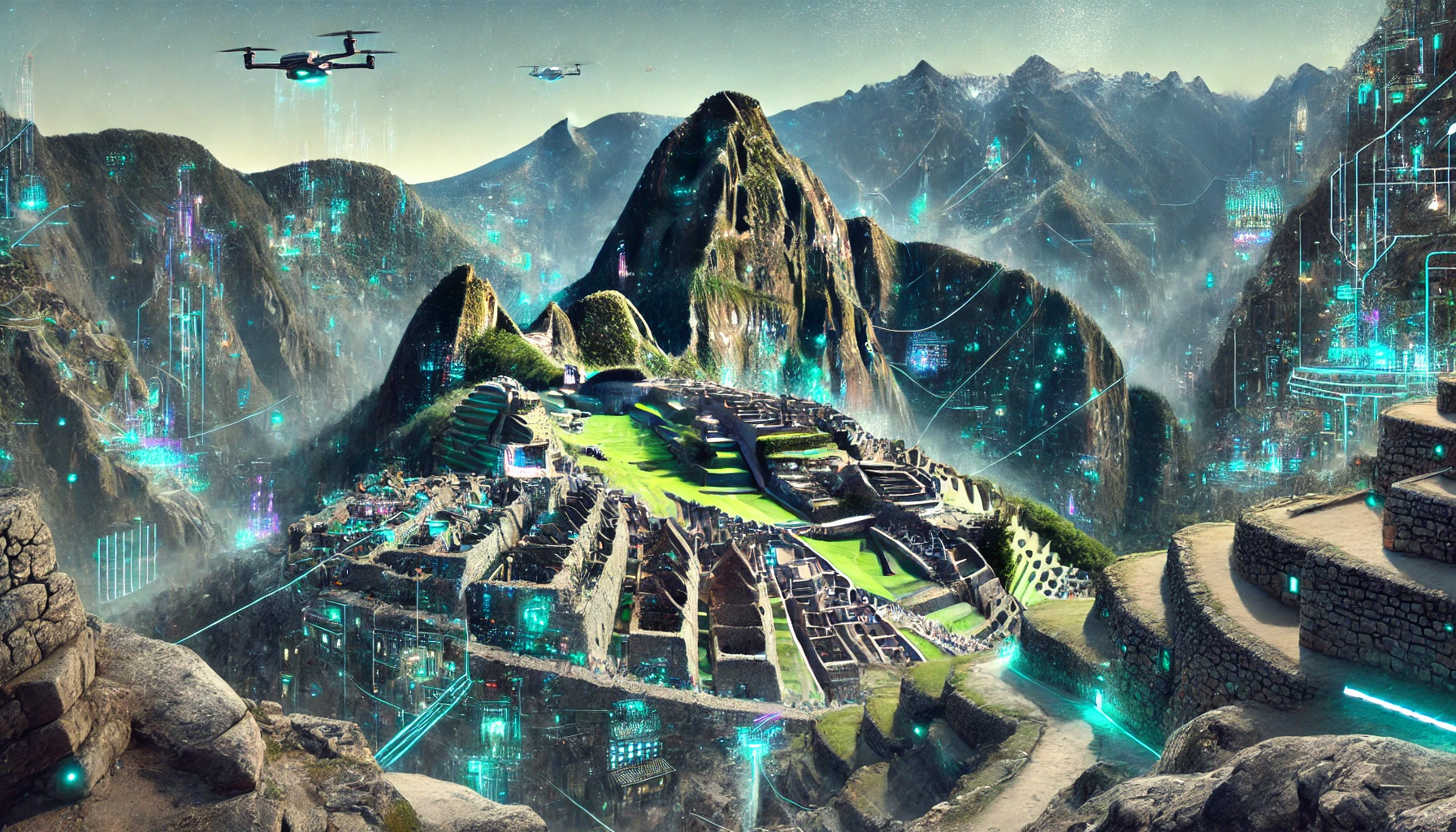
Prompt: Create a futuristic but realistic scenery image of Machu Picchu in Peru, in 1920×1080 resolution. The scene should blend the ancient Incan ruins with futuristic elements like advanced architectural structures integrated into the mountainside, neon-lit pathways, and high-tech transport systems. Include features like solar-powered drones and holographic displays highlighting the ruins. Use a color palette of natural stone colors and greens mixed with vibrant neon accents to create a striking contrast. The composition should evoke a sense of historical depth and futuristic innovation, suitable for a widescreen display, with no text or letters included.
Arequipa
Arequipa is located in southern Peru, in the Andes Mountains. It is the capital of the Arequipa Region and is situated at an altitude of about 2,335 meters (7,661 feet) above sea level. The city lies at the foot of the El Misti volcano, which is one of several volcanoes in the region. Arequipa is approximately 1,000 kilometers (620 miles) southeast of Lima, the capital of Peru. Known for its beautiful colonial architecture made from white volcanic stone, Arequipa is often referred to as “La Ciudad Blanca” or “The White City.”
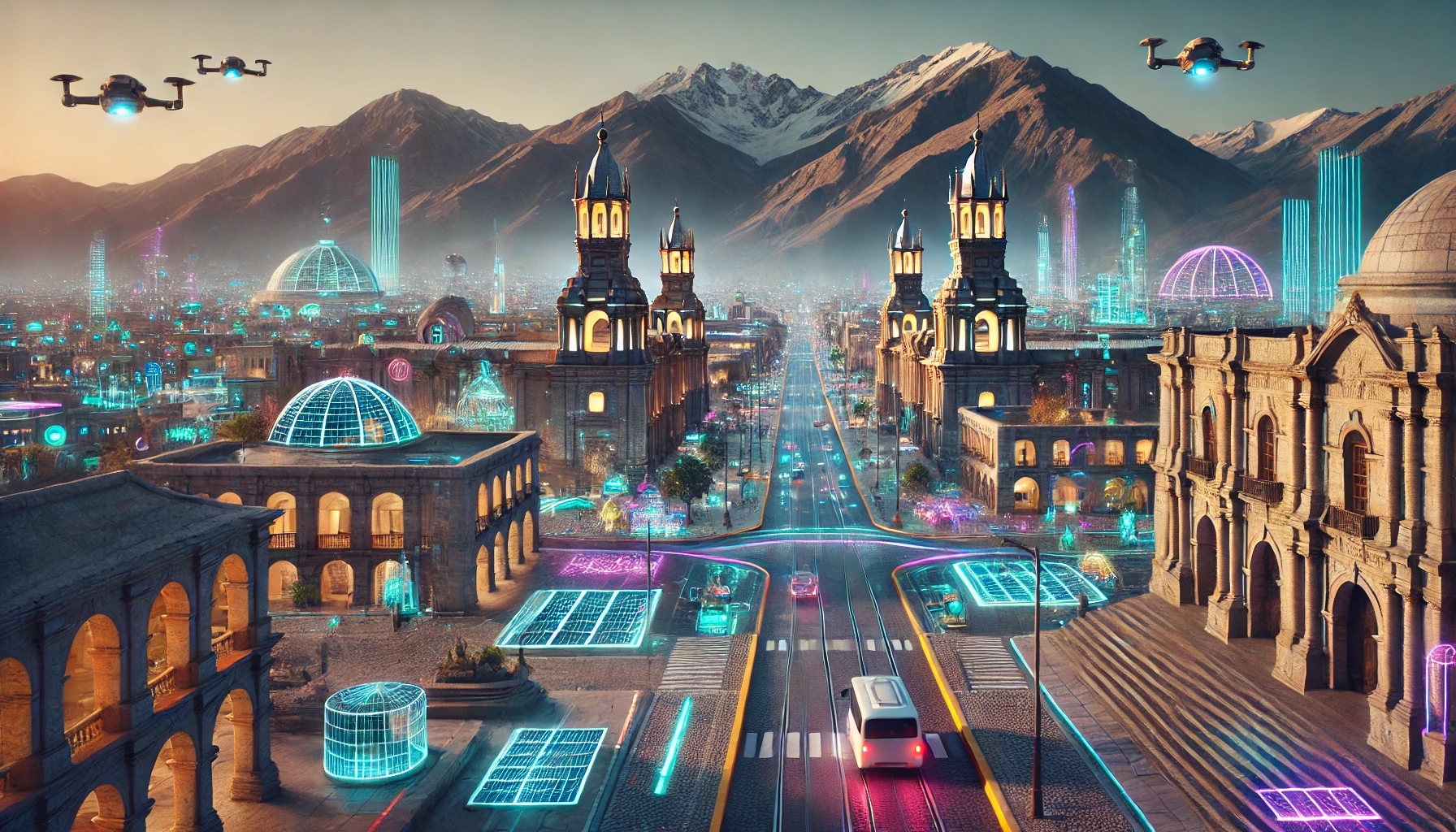
Prompt: Create a futuristic but realistic scenery image of Arequipa, Peru, in 1920×1080 resolution. The scene should blend Arequipa’s iconic colonial architecture and the surrounding Andes mountains with futuristic elements like advanced eco-friendly buildings, neon-lit pathways, and high-tech transportation systems. Include features like solar-powered drones and holographic displays. Use a color palette of natural stone colors and vibrant neon accents to create a striking contrast. The composition should evoke a sense of historical richness and futuristic innovation, suitable for a widescreen display, with no text or letters included.
Amazon Rainforest
The Amazon Rainforest in Peru is located primarily in the eastern part of the country, covering a vast area that includes several regions. The Peruvian Amazon is part of the larger Amazon Basin, which spans several countries in South America.
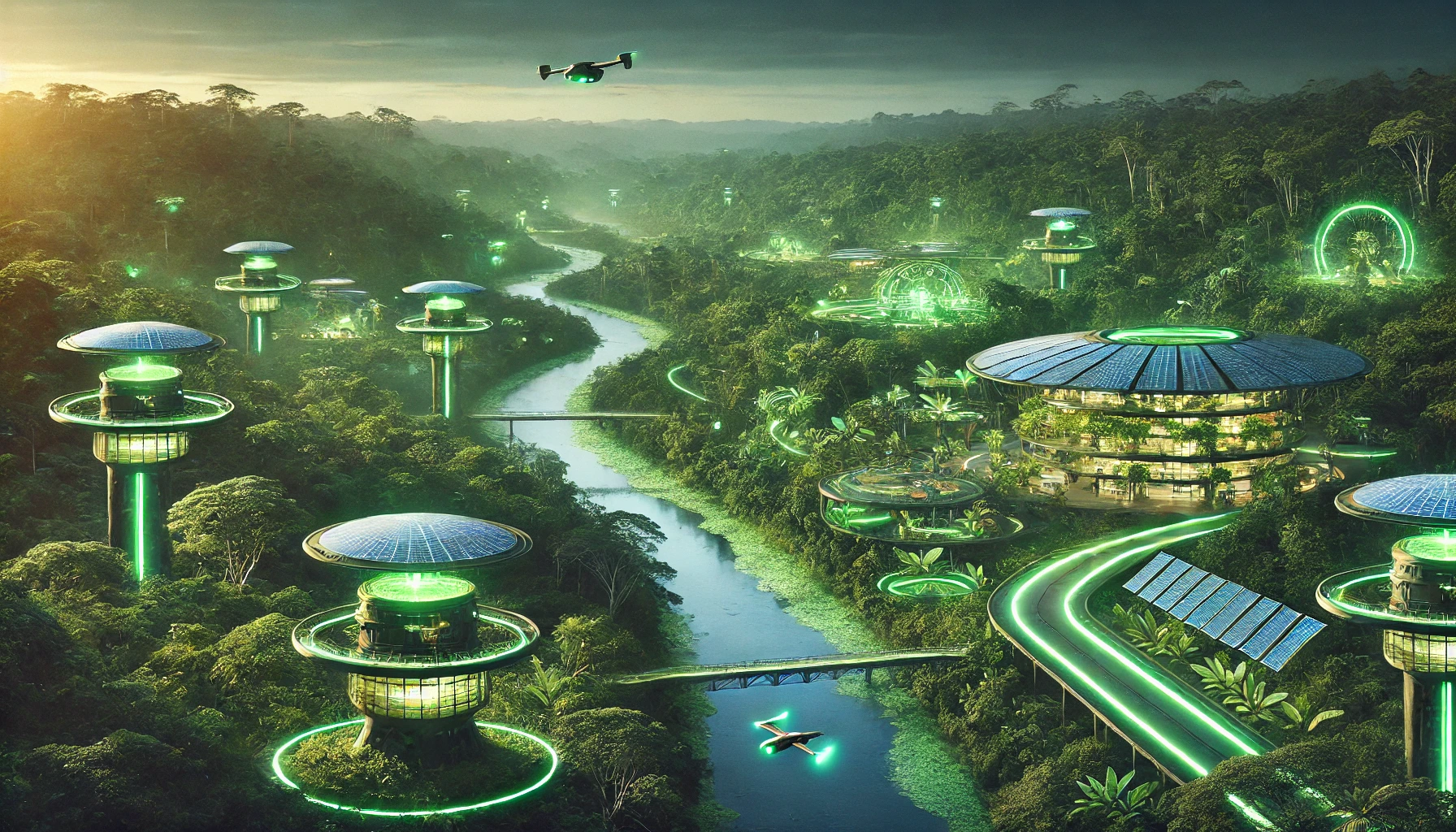
Prompt: Create a futuristic but realistic scenery image of the Amazon Rainforest in Peru, in 1920×1080 resolution. The scene should blend the dense jungle and rich biodiversity of the Amazon with futuristic elements like advanced eco-friendly research stations, neon-lit pathways, and high-tech observation platforms. Include features like solar-powered drones and bioluminescent flora. Use a color palette of vibrant greens and earthy tones mixed with subtle neon accents to create a striking contrast. The composition should evoke a sense of sustainable innovation and futuristic exploration amidst a natural jungle setting, suitable for a widescreen display, with no text or letters included.
Lake Titicaca
The Lake Titicaca is located in the Andes Mountains on the border between Peru and Bolivia. It is situated at an altitude of about 3,812 meters (12,507 feet) above sea level, making it one of the highest navigable lakes in the world. The lake is divided between the southeastern region of Puno in Peru and the western region of La Paz in Bolivia.
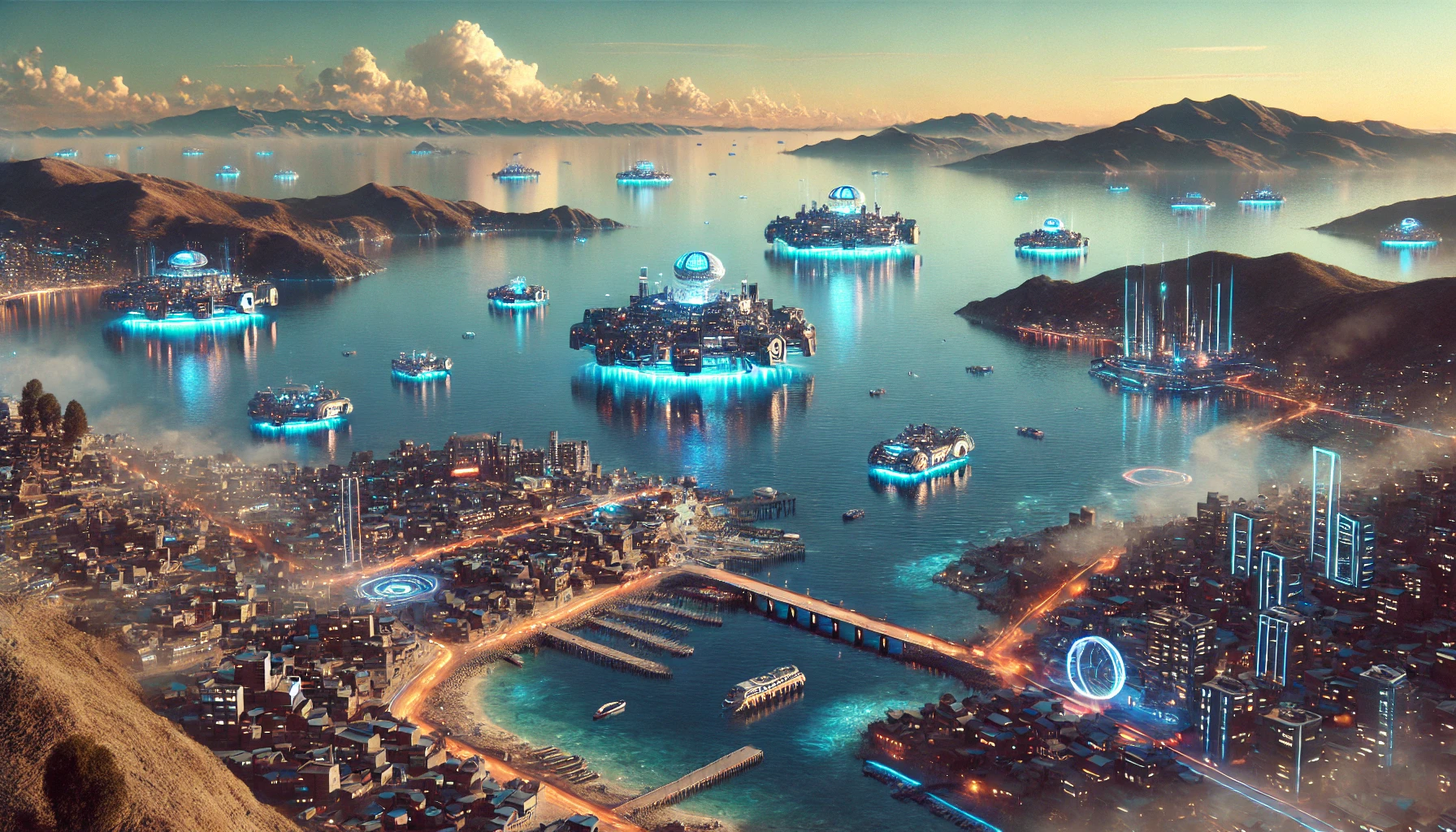
Prompt: Create a futuristic but realistic scenery image of the Lake Titicaca in Peru, in 1920×1080 resolution. The scene should blend the vast, serene waters of Lake Titicaca with futuristic elements like floating cities, neon-lit pathways, and high-tech transportation systems. Include features like hovering boats and holographic displays. Use a color palette of deep blues and earthy tones mixed with vibrant neon colors to create a striking contrast. The composition should evoke a sense of innovative living and futuristic exploration amidst a natural lake setting, suitable for a widescreen display, with no text or letters included.
Rainbow Mountain
The Rainbow Mountain, also known as Vinicunca or Montaña de Siete Colores, is located in the Andes Mountains of Peru, in the Cusco Region. It is situated about 100 kilometers (approximately 62 miles) southeast of the city of Cusco, near the Ausangate Mountain, which is one of the highest peaks in Peru. Rainbow Mountain is known for its vibrant, multicolored slopes, which are a result of mineral deposits that create a unique and stunning landscape. The mountain is a popular destination for hikers and tourists seeking to experience its natural beauty and breathtaking views.
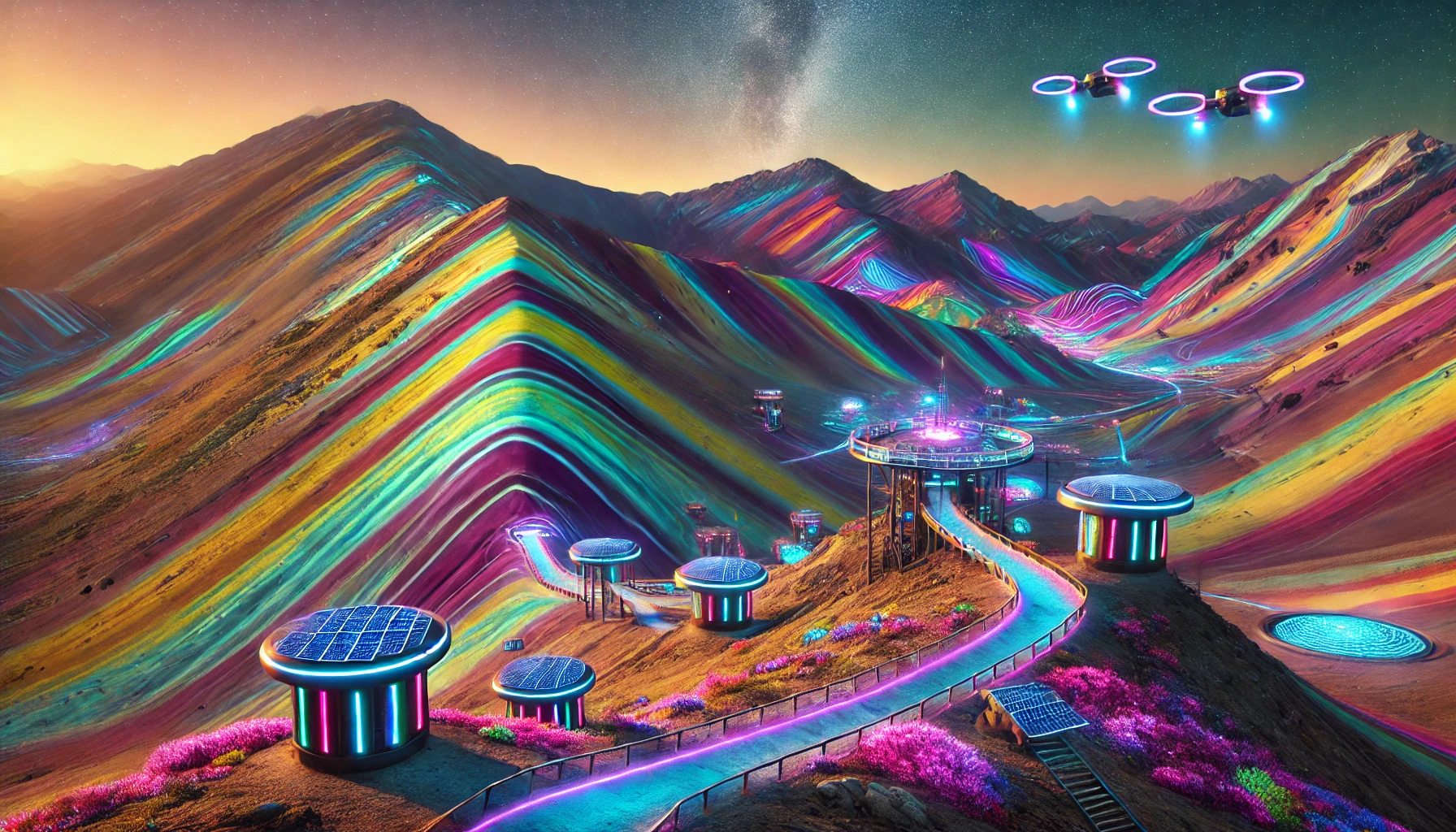
Prompt: Create a futuristic but realistic scenery image of Rainbow Mountain (Vinicunca) in Peru, in 1920×1080 resolution. The scene should blend the vibrant, multicolored slopes of Rainbow Mountain with futuristic elements like advanced observation platforms, neon-lit pathways, and high-tech transportation systems. Include features like solar-powered drones and bioluminescent plants. Use a color palette of natural rainbow hues mixed with vibrant neon accents to create a striking contrast. The composition should evoke a sense of innovative exploration and futuristic wonder amidst a natural setting, suitable for a widescreen display, with no text or letters included.
Nazca Lines
The Nazca Lines are located in the Nazca Desert in southern Peru. They are situated in the Ica Region, approximately 400 kilometers (about 250 miles) south of Lima, the capital city of Peru. The lines are spread over an area of nearly 1,000 square kilometers (about 390 square miles) between the towns of Nazca and Palpa.
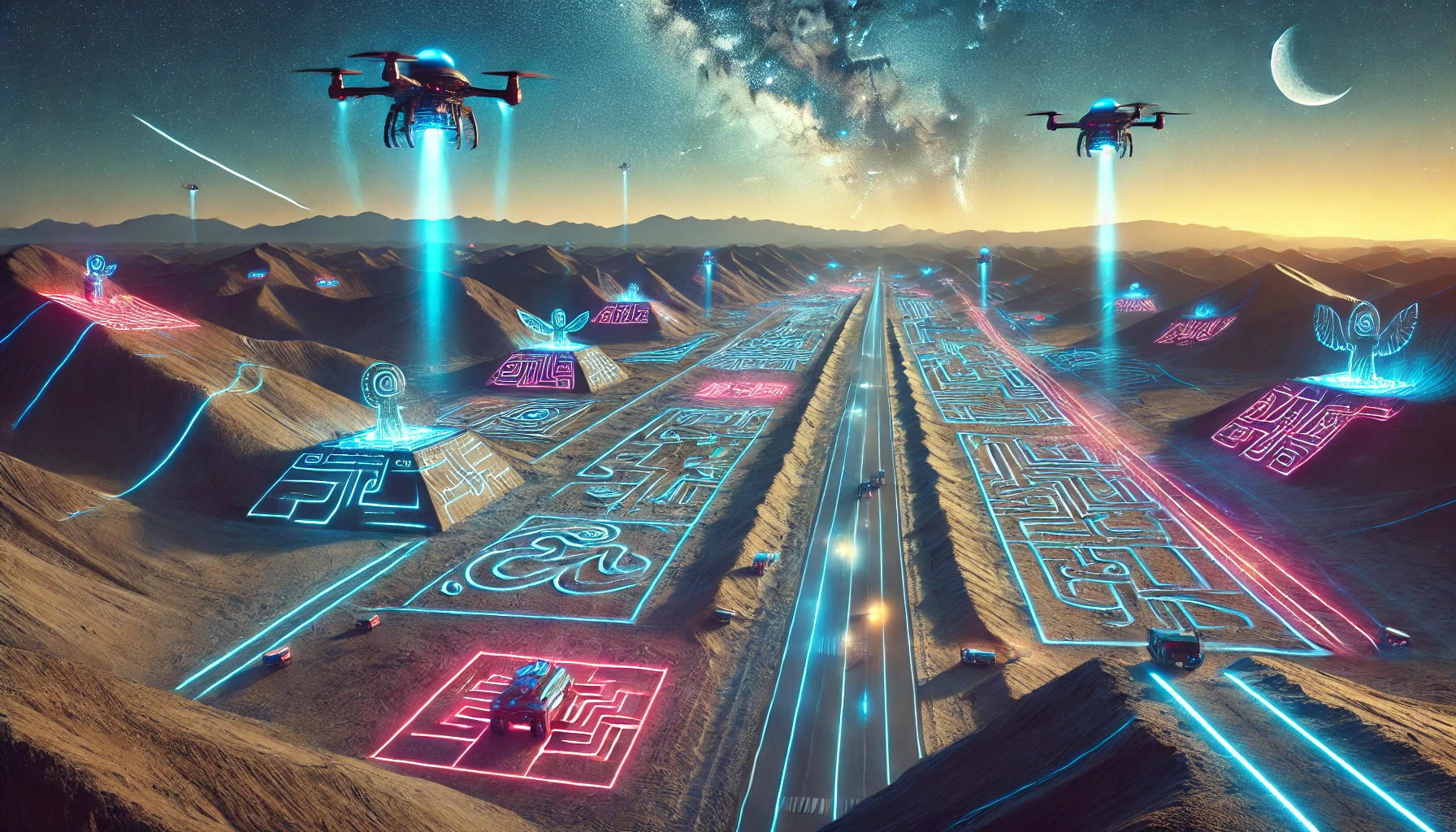
Prompt: Create a futuristic but realistic scenery image of the Nazca Lines in Peru, in 1920×1080 resolution. The scene should blend the iconic geoglyphs of the Nazca Lines with futuristic elements like advanced aerial observation platforms, neon-lit pathways, and high-tech drones flying overhead. Include features like hovering vehicles and holographic displays highlighting the lines. Use a color palette of desert tones mixed with vibrant neon accents to create a striking contrast. The composition should evoke a sense of innovative exploration and futuristic technology amidst a historic setting, suitable for a widescreen display, with no text or letters included.
Sacred Valley
The Sacred Valley, also known as the Urubamba Valley, is located in the Andes Mountains of Peru, near the city of Cusco. It is situated in the Cusco Region and extends from the town of Pisac in the east to Ollantaytambo in the west, following the Urubamba River. The valley is known for its stunning landscapes, fertile lands, and significant archaeological sites, including ancient Incan ruins.
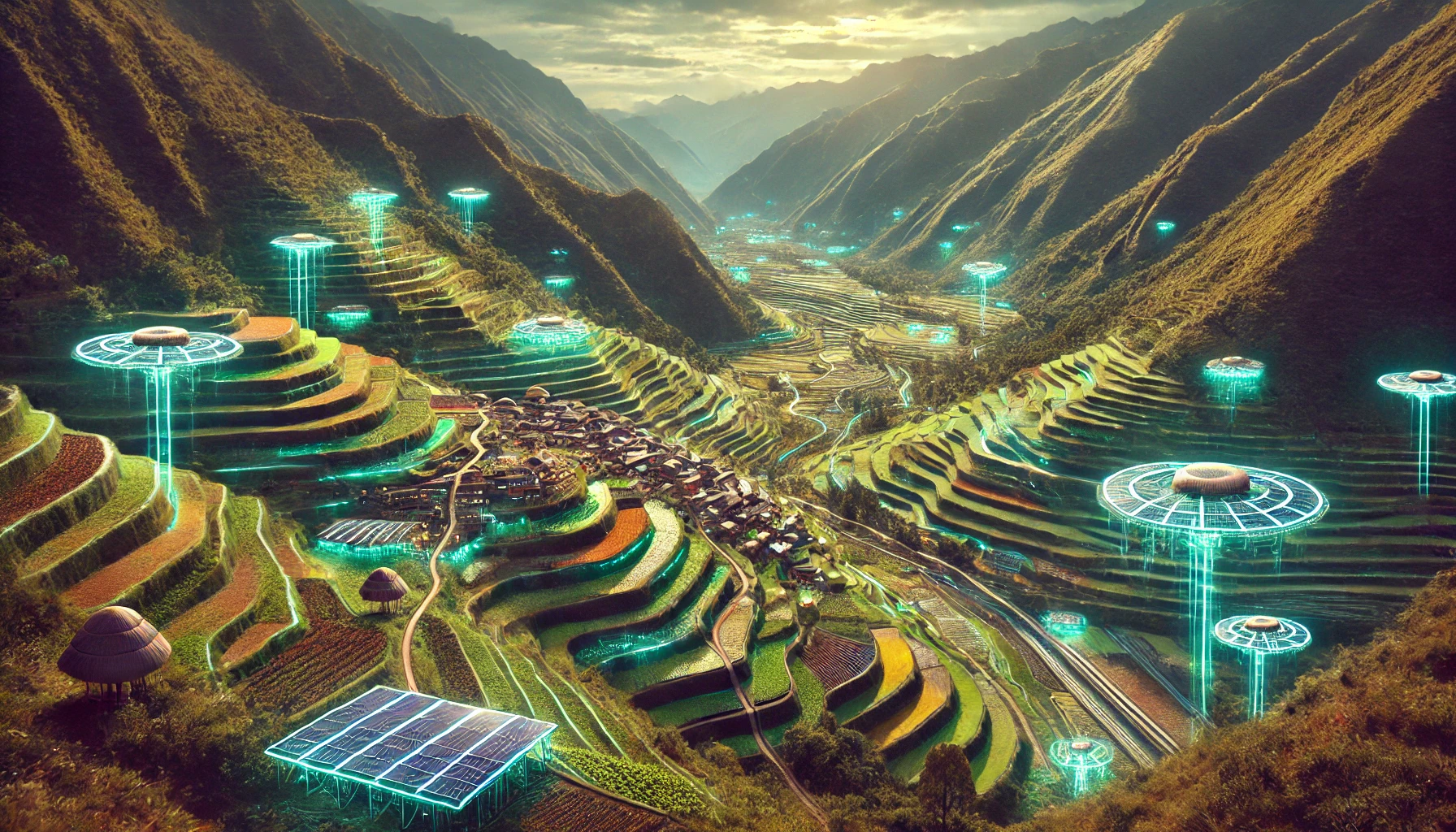
Prompt: Create a futuristic but realistic scenery image of the Sacred Valley in Peru, in 1920×1080 resolution. The scene should blend the lush agricultural terraces and Andean mountains with futuristic elements like advanced eco-friendly infrastructure, neon-lit pathways, and high-tech transportation systems. Include features like solar-powered drones and floating platforms for observation. Use a color palette of natural greens and earthy tones mixed with vibrant neon accents to create a striking contrast. The composition should evoke a sense of historical richness and futuristic innovation, suitable for a widescreen display, with no text or letters included.
Huacachina Oasis
The Huacachina Oasis is located in southwestern Peru, near the city of Ica. It is situated about 8 kilometers (5 miles) west of the city center of Ica and approximately 300 kilometers (186 miles) south of Lima, the capital of Peru.
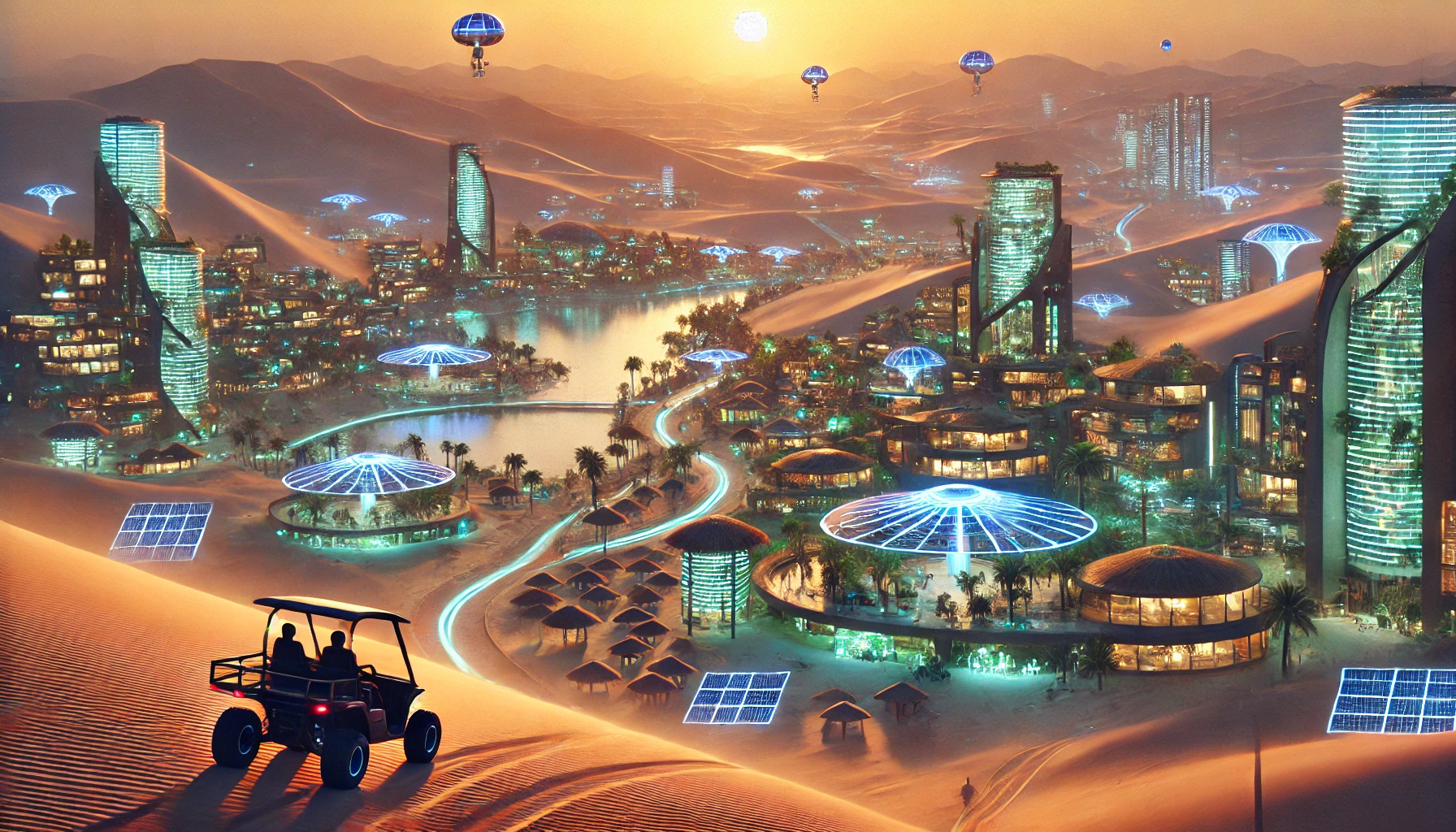
Prompt: Create a futuristic but realistic scenery image of Huacachina in Peru, in 1920×1080 resolution. The scene should blend the iconic desert oasis with futuristic elements like advanced eco-friendly resorts, neon-lit pathways, and high-tech transportation systems. Include features like solar-powered dune buggies and floating observation platforms. Use a color palette of natural desert hues mixed with vibrant neon accents to create a striking contrast. The composition should evoke a sense of adventurous exploration and futuristic innovation amidst a natural desert setting, suitable for a widescreen display, with no text or letters included.
Paracas National Reserve
The Paracas National Reserve is located on the southern coast of Peru, in the Ica Region. It is situated approximately 250 kilometers (about 155 miles) south of Lima, the capital city of Peru, and is near the town of Paracas.
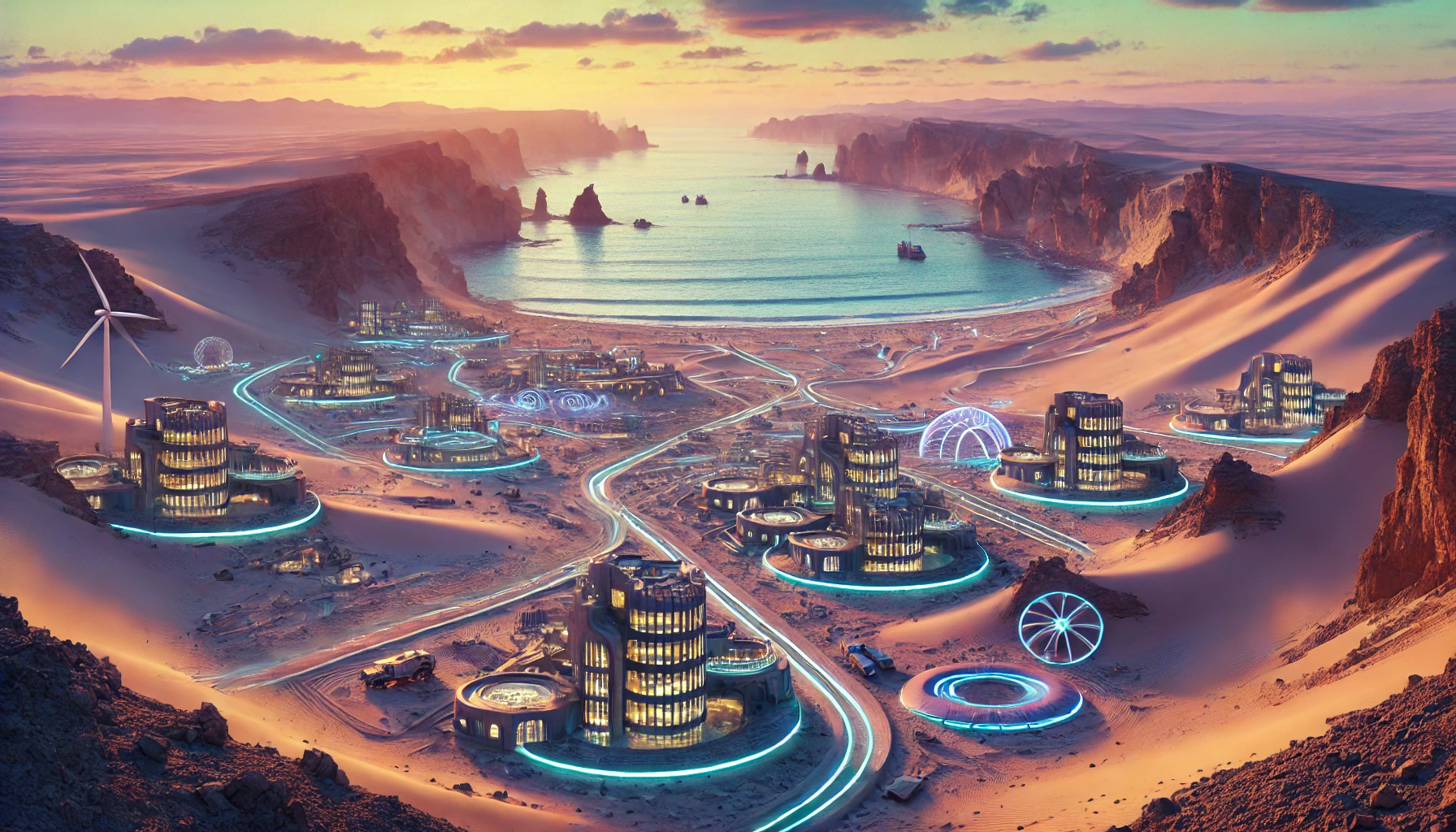
Prompt: Create a futuristic but realistic scenery image of the Paracas National Reserve in Peru, in 1920×1080 resolution. The scene should blend the stunning desert landscapes and coastal cliffs of the Paracas National Reserve with futuristic elements like advanced eco-friendly habitats, neon-lit pathways, and high-tech transportation systems. Include features like solar-powered vehicles and wind energy structures. Use a color palette of natural desert and ocean tones mixed with subtle neon accents to create a striking contrast. The composition should evoke a sense of sustainable innovation and futuristic life amidst a natural setting, suitable for a widescreen display, with no text or letters included.
Tampopata National Reserve
The Tambopata National Reserve is located in the southeastern part of Peru, within the Madre de Dios Region. It is situated in the Amazon Basin, near the border with Bolivia and Brazil. The reserve is accessible from the city of Puerto Maldonado, which is the capital of the Madre de Dios Region and serves as a gateway to the reserve.
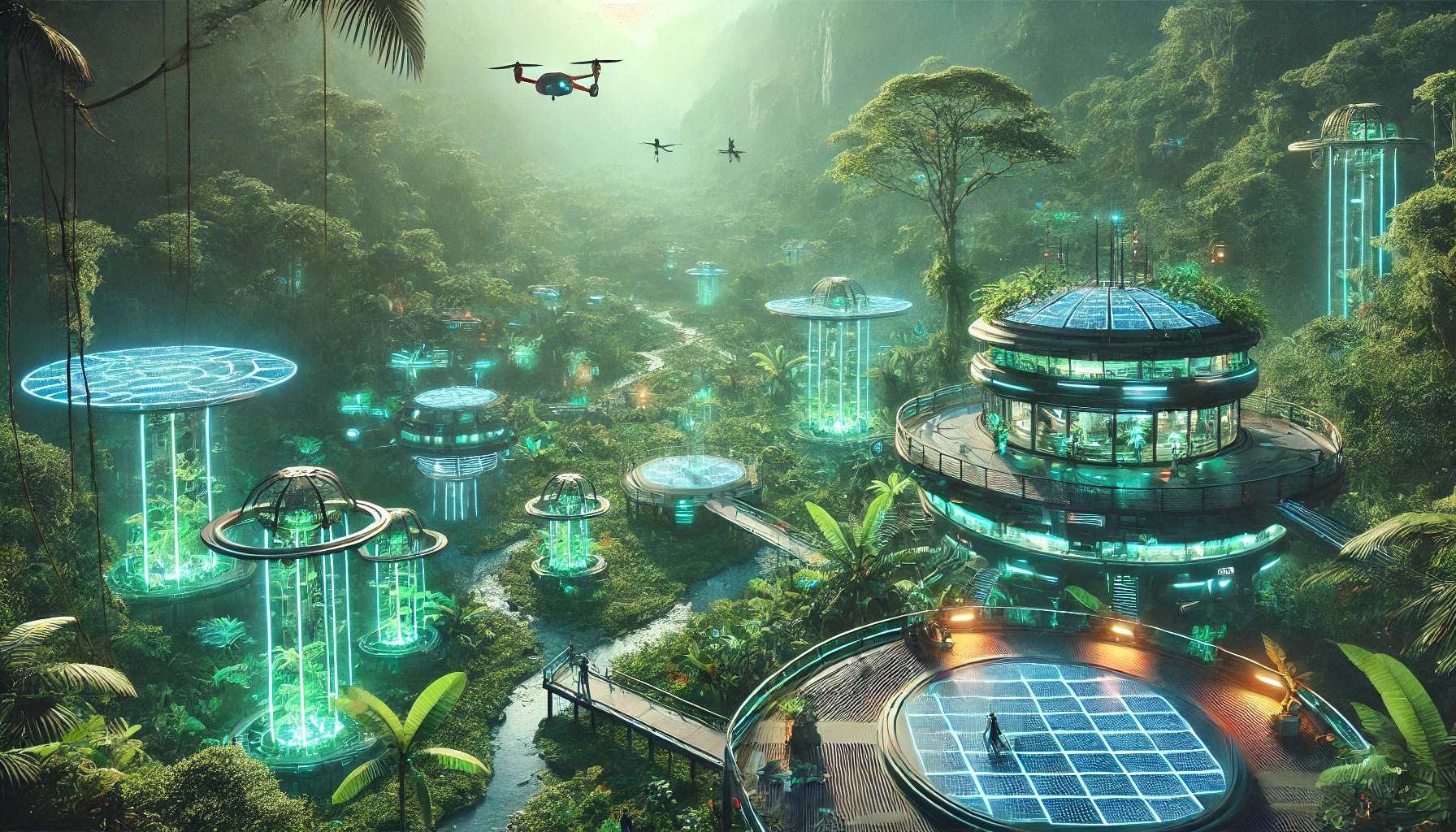
Prompt: Create a futuristic but realistic scenery image of Tambopata National Reserve in Peru, in 1920×1080 resolution. The scene should blend the lush rainforest and diverse wildlife of Tambopata with futuristic elements like advanced eco-friendly research stations, neon-lit pathways, and high-tech observation platforms. Include features like solar-powered drones and bioluminescent plants. Use a color palette of vibrant greens and earthy tones mixed with subtle neon accents to create a striking contrast. The composition should evoke a sense of sustainable innovation and futuristic life amidst a natural jungle setting, suitable for a widescreen display, with no text or letters included.
Colca Canyon
Colca Canyon is located in southern Peru, in the Arequipa Region. It is situated approximately 160 kilometers (about 100 miles) northwest of the city of Arequipa. The canyon is one of the deepest in the world, with a depth of more than 3,270 meters (10,725 feet) at its deepest point.
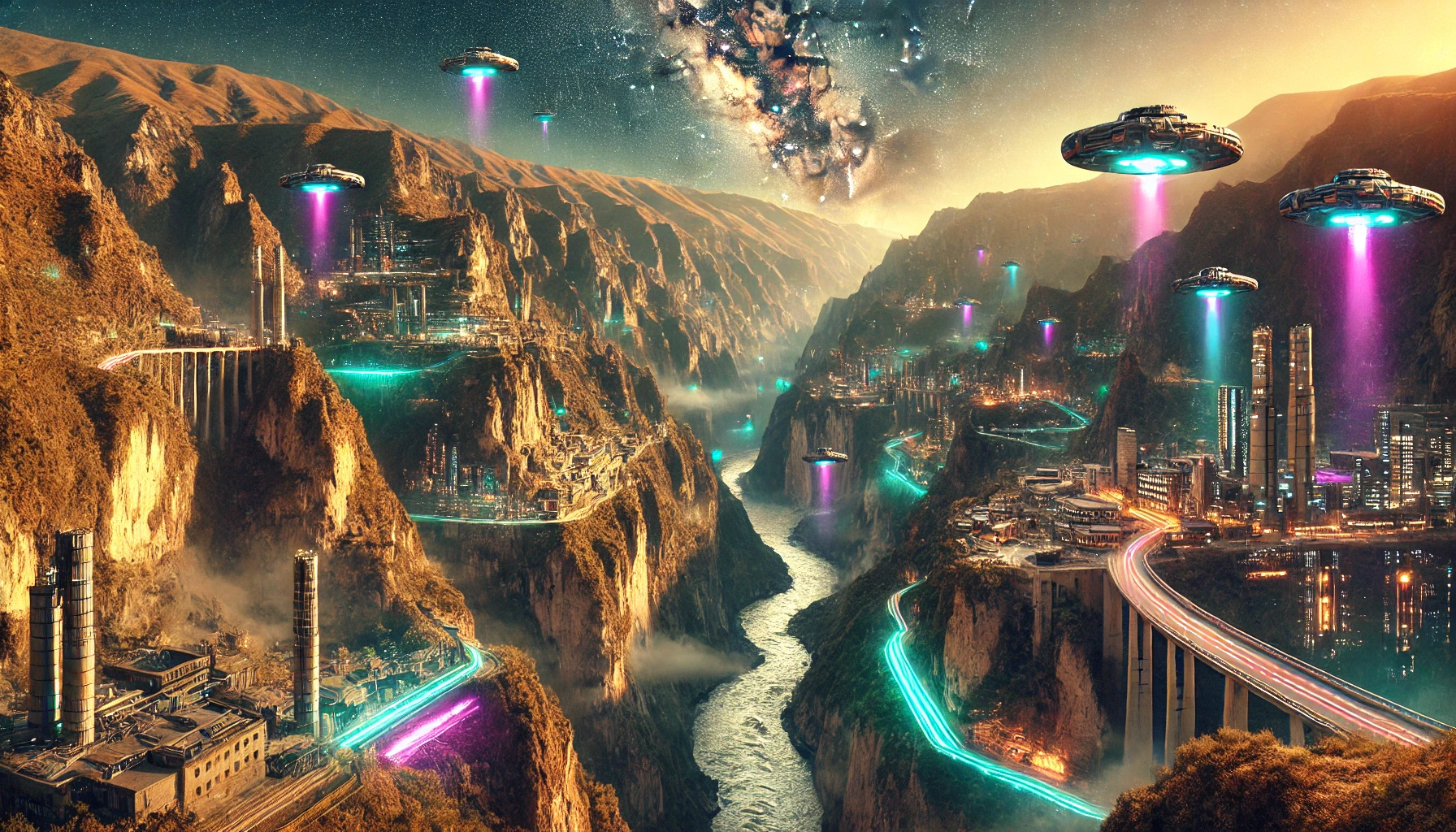
Prompt: Create a futuristic scenery image of Colca Canyon in Peru, inspired by science fiction themes in 1920×1080 resolution. The scene should blend the dramatic landscapes and deep ravines of Colca Canyon with futuristic elements like advanced architecture integrated into the cliffs, neon-lit pathways, and high-tech transportation systems. Include features like hovering vehicles and bioluminescent flora. Use a color palette of natural earth tones and vibrant neon colors to create a striking contrast. The composition should evoke a sense of innovation and futuristic life amidst a rugged and natural setting, suitable for a widescreen display, with no text or letters included.
Final Thoughts
The process of generating futuristic images of Peru not only highlights the creative potential of AI but also underscores the importance of prompt engineering in achieving the desired visual outcomes. Effective prompting techniques involve iterative experimentation, where precise instructions are crafted to guide the AI in blending disparate elements like historical sites and futuristic technology. As AI continues to advance, we can expect improvements in model accuracy, allowing for even more nuanced interpretations and seamless integrations of complex concepts. This evolution will likely be driven by enhanced AI architectures, better training datasets, and more sophisticated prompt crafting methodologies.
Looking ahead, image generation tools are poised to become increasingly interactive and intuitive, empowering users to create even more personalized and contextually rich visual narratives. These tools could offer real-time feedback, allowing creators to make dynamic adjustments and achieve optimal results efficiently. The ongoing development of AI-driven creativity tools presents an exciting frontier, opening new possibilities for artists, designers, and innovators to explore and redefine the boundaries of art and technology. As we conclude this exploration, it is clear that AI will play a pivotal role in shaping the future of visual storytelling, fostering a harmonious blend of tradition and innovation that can inspire and captivate audiences worldwide.
Follow me on Twitter and LinkedIn for new content on generative AI. Check out Generative AI Lab for some experiments. Last but not least, join Learn AI Together by Towards AI and let’s explore the world of AI together.
Resource recommendations to get started with generative AI: