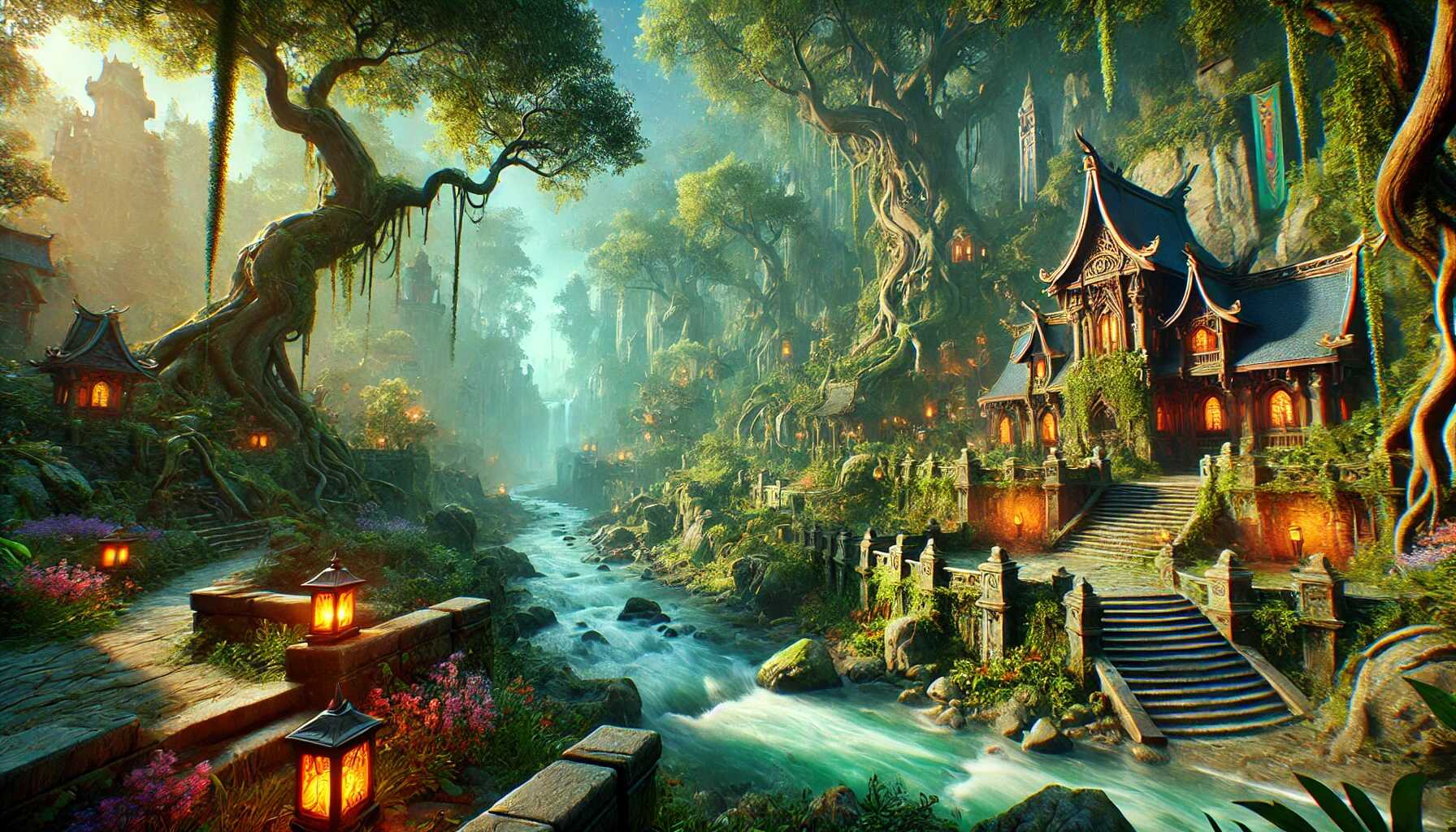

TL;DR: This blog post explores the top books on generative AI, covering a wide range of topics from foundational concepts to practical applications. Whether you’re a developer or enthusiast, these books offer valuable insights into the world of generative AI. The recommended books include practical guides to building generative AI models, understanding their ethical implications, and leveraging them for business applications.
Introduction
The field of Generative AI is rapidly evolving, with new breakthroughs and applications emerging daily. To stay updated on the latest advancements, books offer a structured and in-depth approach to understanding these complex topics. This blog post will explore some of the top books on Generative AI, providing a brief overview, table of contents, and Amazon links for each.
Generative Deep Learning: Teaching Machines To Paint, Write, Compose, and Play
TL;DR: Generative Deep Learning: Teaching Machines To Paint, Write, Compose, and Play is a comprehensive guide to building and training generative AI models using TensorFlow and Keras. It covers a wide range of techniques, from VAEs and GANs to Transformers and diffusion models. The book is ideal for machine learning engineers and data scientists looking to explore the exciting world of generative AI.
Table of Contents:
- Part I: Introduction to Generative Deep Learning
- What is Generative Deep Learning?
- A Brief History of Generative Models
- Deep Learning Fundamentals
- Part II: Variational Autoencoders (VAEs)
- Understanding VAEs
- Building and Training VAEs
- Applications of VAEs
- Part III: Generative Adversarial Networks (GANs)
- Understanding GANs
- Building and Training GANs
- Applications of GANs
- Part IV: Transformers
- Understanding Transformers
- Building and Training Transformers
- Applications of Transformers
- Part V: Normalizing Flows
- Understanding Normalizing Flows
- Building and Training Normalizing Flows
- Applications of Normalizing Flows
- Part VI: Energy-Based Models
- Understanding Energy-Based Models
- Building and Training Energy-Based Models
- Applications of Energy-Based Models
- Part VII: Denoising Diffusion Models
- Understanding Denoising Diffusion Models
- Building and Training Denoising Diffusion Models
- Applications of Denoising Diffusion Models
- Part VIII: The Future of Generative AI
- Trends and Predictions
- Ethical Considerations
- Opportunities and Challenges
Prompt Engineering for Generative AI: Future-Proof Inputs for Reliable AI Outputs 1st Edition
TL;DR: Prompt Engineering for Generative AI: Future-Proof Inputs for Reliable AI Outputs is a practical guide to effectively interacting with Large Language Models (LLMs) and diffusion models. The book covers the principles of prompt engineering, which can help you coax reliable results from these models. It’s essential reading for developers looking to harness the power of generative AI.
Table of Contents:
- Understanding the Interaction Chain
- The Role of Prompts
- The Structure of the Interaction Chain
- Transforming Problems into Document Completion
- Problem Formulation
- Prompt Design
- Leveraging LLM and Diffusion Model Architecture
- Model Selection
- Prompt Optimization
- Prompt Engineering in Practice
- Natural Language Processing
- Text and Image Generation
- Code Generation
Generative AI on AWS: Building Context-Aware Multimodal Reasoning Applications 1st Edition
TL;DR: Generative AI on AWS: Building Context-Aware Multimodal Reasoning Applications is a practical guide to implementing generative AI in your business. It covers the entire project life cycle, from use case definition to deployment. The book explores different types of generative AI models, including LLMs and multimodal models, and provides guidance on techniques like prompt engineering, fine-tuning, and reinforcement learning.
Table of Contents:
- Understanding Generative AI
- What is Generative AI?
- Types of Generative AI Models
- The Generative AI Project Lifecycle
- Use Case Definition
- Model Selection
- Model Fine-tuning
- Retrieval-Augmented Generation
- Reinforcement Learning from Human Feedback
- Model Quantization, Optimization, and Deployment
- Building Context-Aware Applications
- Prompt Engineering
- In-Context Learning
- LLMs and Multimodal Models
- Leveraging AWS Services
- Amazon Bedrock
- SageMaker
- Other Relevant Services
- Case Studies and Best Practices
- Real-world Examples
- Tips and Tricks
Generative AI with LangChain: Build large language model (LLM) apps with Python, ChatGPT and other LLMs
TL;DR: Generative AI with LangChain: Build large language model (LLM) apps with Python, ChatGPT and other LLMs is a practical guide to building LLM applications using the LangChain framework. It covers the fundamentals of LLMs, prompt engineering, fine-tuning, and deployment. The book is ideal for developers looking to harness the power of generative AI.
Table of Contents:
- Understanding LLMs
- What are LLMs?
- Strengths and Limitations
- Generative AI Fundamentals
- Key Concepts
- Industry Trends
- Getting Started with LangChain
- Installation and Setup
- Basic Usage
- Building Capable Assistants
- Question-Answering Systems
- Chatbots
- Developing Software with Generative AI
- Code Generation
- Data Analysis
- Customizing LLMs
- Prompt Engineering
- Fine-tuning
- Generative AI in Production
- Deployment Strategies
- Evaluation
- The Future of Generative Models
- Trends and Predictions
Generative AI with Python and TensorFlow 2: Create images, text, and music with VAEs, GANs, LSTMs, Transformer models
TL;DR: Generative AI with Python and TensorFlow 2: Create images, text, and music with VAEs, GANs, LSTMs, Transformer models is a practical guide to building generative AI models using TensorFlow 2. It covers a wide range of techniques and applications, from text generation to image manipulation. The book is ideal for Python programmers interested in exploring the creative possibilities of generative AI.
Table of Contents:
- Introduction to Generative AI
- What is Generative AI?
- History of Generative Models
- Deep Learning Fundamentals
- TensorFlow 2 Basics
- Neural Networks
- Variational Autoencoders (VAEs)
- Understanding VAEs
- Building VAEs with TensorFlow
- Generative Adversarial Networks (GANs)
- Understanding GANs
- Building GANs with TensorFlow
- Long Short-Term Memory (LSTM) Networks
- Understanding LSTMs
- Building LSTMs with TensorFlow
- Transformer Models
- Understanding Transformers
- Building Transformers with TensorFlow
- Applications of Generative AI
- Text Generation
- Image Generation
- Music Generation
- Other Applications
TL;DR: Introduction to Generative AI is a comprehensive guide to understanding and using generative AI tools like ChatGPT. It covers the basics of LLMs, best practices for using these tools, and the ethical considerations surrounding their use. The book is ideal for anyone interested in learning more about generative AI.
Table of Contents:
- Large Language Models
- Evolution of NLP
- Training LLMs
- Data Privacy and Safety
- Data Privacy Concerns
- Mitigating Risks
- Created Content
- Impact on Content Creation
- Ethical Implications
- Misuse and Adversarial Attacks
- Identifying and Preventing Misuse
- Accelerating Productivity
- Using AI for Work
- Social Connections with Chatbots
- Building Relationships with AI
- The Future of AI
- Trends and Predictions
Generative AI for Cloud Solutions: Architect modern AI LLMs in secure, scalable, and ethical cloud environments
TL;DR: Generative AI for Cloud Solutions: Architect modern AI LLMs in secure, scalable, and ethical cloud environments is a comprehensive guide to implementing generative AI, specifically LLMs and ChatGPT, on cloud platforms. It covers topics like fine-tuning, prompt engineering, responsible AI, and scaling strategies. The book is ideal for cloud architects, data scientists, and AI researchers looking to harness the power of generative AI in the cloud.
Table of Contents:
- Cloud Computing Meets Generative AI
- Introduction to Cloud Computing
- Introduction to Generative AI
- NLP Evolution and Transformers
- Understanding NLP
- Transformers and LLMs
- Fine-Tuning LLMs
- Customizing LLMs for Specific Tasks
- Retrieval-Augmented Generation (RAG)
- Enhancing LLM Performance with External Information
- Prompt Engineering
- Crafting Effective Prompts
- Developing LLM-Based Cloud Applications
- Frameworks and Tools
- LLMOps
- Deploying ChatGPT in the Cloud
- Architecture Design
- Scaling Strategies
- Security and Privacy
- Protecting Data and Models
- Responsible AI
- Ethical Considerations
- Future of Generative AI
- Trends and Emerging Use Cases
Generative AI: Navigating the Course to the Artificial General Intelligence Future 1st Edition
TL;DR: Generative AI: Navigating the Course to the Artificial General Intelligence Future is a comprehensive exploration of generative AI by Martin Musiol. It covers the history, current capabilities, and future potential of generative AI, including its role in the development of Artificial General Intelligence (AGI). The book also explores the ethical considerations and practical applications of AI assistants.
Table of Contents:
- Introduction to Generative AI
- What is Generative AI?
- A Brief History of Generative Models
- Understanding Generative AI Techniques
- Variational Autoencoders (VAEs)
- Generative Adversarial Networks (GANs)
- Other Generative AI Models
- The Road to Artificial General Intelligence
- How Generative AI Contributes to AGI
- Merging Current AI Models
- The Ethical Landscape of AI
- Potential Downsides of AI
- Responsible Development of AI
- Autonomous AI Agents and the Future of Work
- How AI Assistants will Transform Our Lives
- Applications in Different Fields
The ChatGPT Millionaire: Making Money Online has never been this EASY (Chat GPT and Generative AI Mastery Series)
TL;DR: The ChatGPT Millionaire: Making Money Online has never been this EASY is a guide to using ChatGPT to generate income online. It covers topics like creating passive income sources, writing engaging content, and automating tasks. The book includes practical examples and a bonus ChatGPT prompt cheat sheet.
Table of Contents:
- Introduction to ChatGPT
- What is ChatGPT?
- How Does it Work?
- Making Money with ChatGPT
- Creating Passive Income Sources
- Writing Engaging Content
- Automating Tasks
- Effective ChatGPT Usage
- Guidelines and Tips
- ChatGPT Prompts
- 150+ Prompts for Various Tasks
- Limitations of ChatGPT
- Understanding ChatGPT’s Capabilities
The field of Generative AI is rapidly evolving, offering exciting possibilities for developers, artists, and anyone curious about the future of technology. This blog post has explored some of the best books on Generative AI, providing a roadmap to enhance your understanding of this transformative field.
Whether you’re a seasoned developer seeking to build generative models or a complete beginner intrigued by the potential of AI-powered content creation, these books cater to a wide range of audiences. From foundational concepts like Variational Autoencoders (VAEs) and Generative Adversarial Networks (GANs) to practical applications in areas like text generation and image manipulation, these resources offer valuable insights.
Keep in mind: This list is not exhaustive, and there are many other excellent books available. Consider your specific interests and goals when choosing a book to delve deeper into the world of Generative AI.
Do you have a book suggestion to add to this list of the best books on large language models? Please send it to us. We’d love to hear from you.
Join us on this incredible generative AI journey and be a part of the revolution. Stay tuned for updates and insights on generative AI by following us on X or LinkedIn.