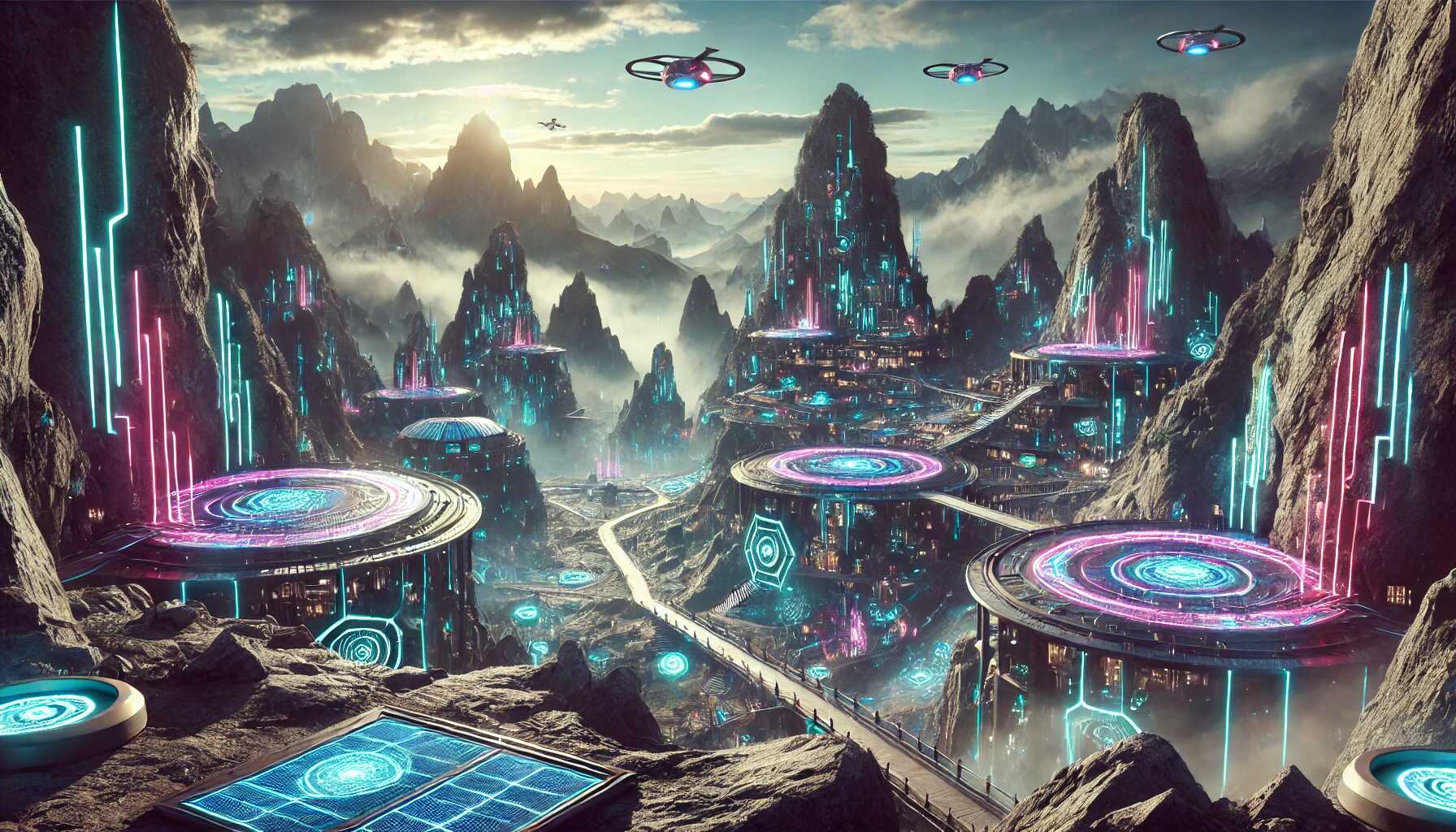
Author(s): Daniel Pollak

TL;DR: Heckman Selection Bias Modeling is a statistical technique used in causal studies to account for the bias caused by non-random selection of participants. It involves estimating the likelihood of selection and adjusting the results accordingly. This approach helps to provide more accurate and reliable results in research studies.
Disclaimer: This post has been created automatically using generative AI. Including DALL-E, Gemini, OpenAI and others. Please take its contents with a grain of salt. For feedback on how we can improve, please email us
Introduction to Heckman Selection Bias Modeling
Causal studies are an essential tool for understanding the relationships between different variables and determining cause and effect. However, these studies can be impacted by selection bias, which occurs when certain factors influence the selection of participants in a study. This can lead to biased results and incorrect conclusions. One method that has been developed to address this issue is the Heckman Selection Bias Model. In this blog post, we will explore the concept of selection bias and how the Heckman Model can help address it in causal studies.
Understanding Selection Bias in Causal Studies
Selection bias occurs when certain factors influence the selection of participants in a study, and this can have a significant impact on the results. For example, if a study only includes participants from a specific demographic, the results may not be applicable to the general population. Similarly, if participants self-select to participate in a study, they may have different characteristics than those who do not participate, leading to biased results. Selection bias can also occur when participants drop out of a study before it is completed, leading to incomplete data and potentially skewed results.
Introduction to the Heckman Selection Bias Model
The Heckman Selection Bias Model was developed by economist James Heckman to address the issue of selection bias in causal studies. The model uses a two-step approach to account for selection bias. In the first step, a probit regression model is used to estimate the probability of selection into the study. This model takes into account the characteristics of the participants and the factors that may have influenced their selection. In the second step, the model uses the inverse Mills ratio, which is derived from the probit regression, to adjust the outcome variable and account for selection bias.
Advantages of the Heckman Selection Bias Model
The Heckman Selection Bias Model has several advantages over other methods of dealing with selection bias. One of the main advantages is that it allows for the inclusion of both observed and unobserved variables in the analysis. This means that factors that may have influenced the selection of participants, but were not measured, can still be accounted for in the model. Additionally, the model can handle different types of selection bias, such as sample selection, attrition, and non-response bias. This makes it a versatile tool for addressing selection bias in various types of studies.
Limitations of the Heckman Selection Bias Model
In conclusion, the use of Heckman selection bias modeling in causal studies has proven to be a valuable tool for addressing potential bias and improving the accuracy of causal inference. By accounting for selection bias, researchers are able to obtain more reliable and valid estimates of causal effects. This approach has the potential to enhance the quality of research findings and ultimately contribute to better-informed decision making. Overall, the application of Heckman selection bias modeling has greatly advanced the field of causal studies and continues to be a relevant and valuable method for future research.
Crafted using generative AI from insights found on Towards Data Science.
Join us on this incredible generative AI journey and be a part of the revolution. Stay tuned for updates and insights on generative AI by following us on X or LinkedIn.