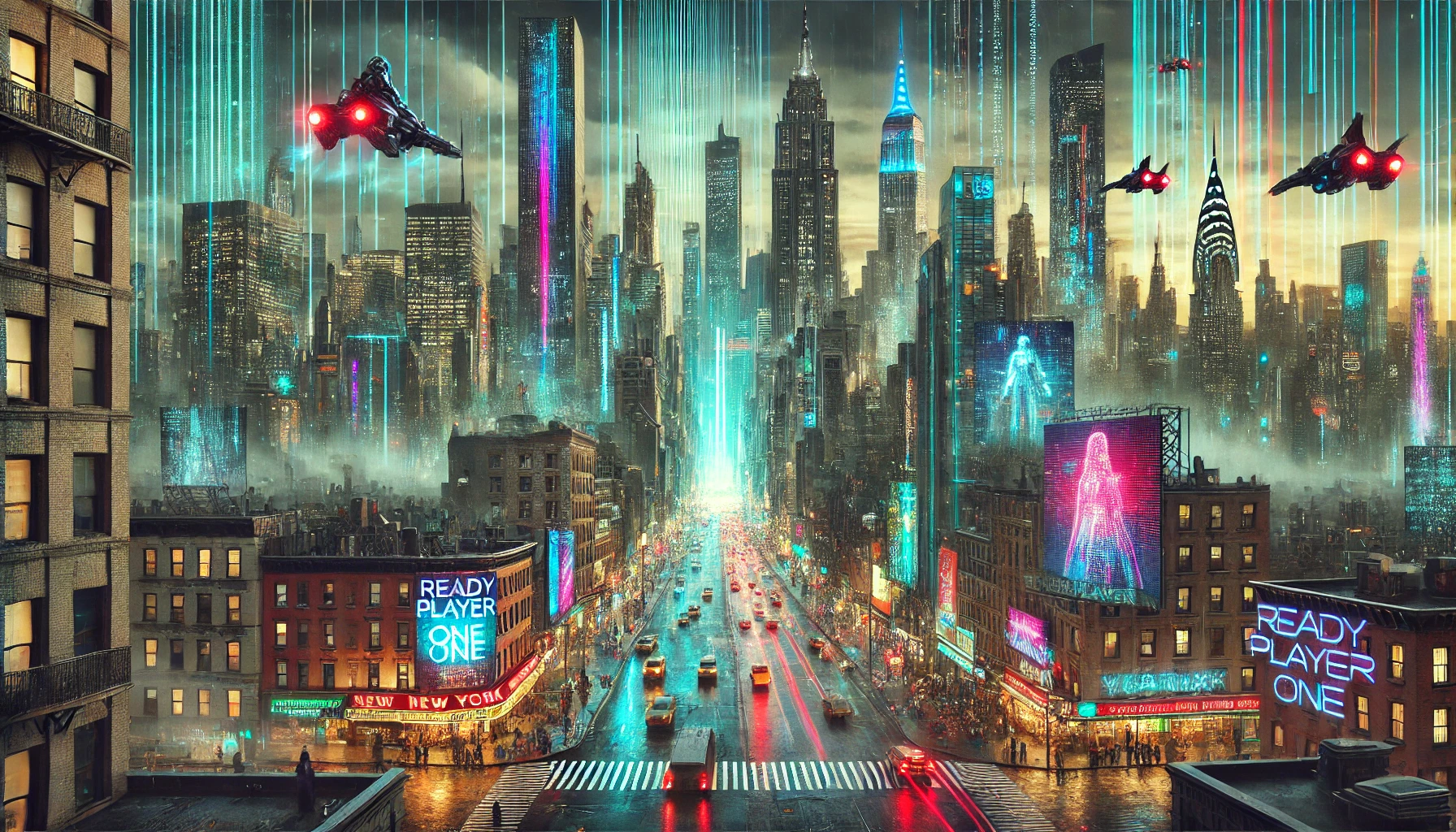
TL;DR: Quantile loss, also known as the pinball loss, is a way to evaluate probabilistic forecasts. It measures the difference between the actual and predicted values at different probability levels, allowing for a more nuanced understanding of forecast accuracy. This metric is commonly used in fields such as finance and weather forecasting.
Disclaimer: This post has been created automatically using generative AI. Including DALL-E, and OpenAI. Please take its contents with a grain of salt. For feedback on how we can improve, please email us
Introduction
In the world of data science and forecasting, it is important to have metrics that can accurately evaluate the performance of probabilistic forecasts. One such metric is the quantile loss, also known as the pinball loss. In this blog post, we will introduce the concept of quantile loss and discuss the intuition behind this metric.
What is Quantile Loss?
Quantile loss is a metric used to evaluate the accuracy of probabilistic forecasts. It measures the difference between the predicted quantiles and the actual values of a variable. In simpler terms, it measures how well a forecast captures the uncertainty in the data. The lower the quantile loss, the better the forecast.
The Pinball Analogy
The term “pinball loss” comes from the analogy of a pinball machine. In a pinball machine, the ball bounces around and can end up in different locations. Similarly, in probabilistic forecasting, the actual value of a variable can fall anywhere within the predicted range. The quantile loss measures the distance between the actual value and the predicted quantiles, just like the distance between the ball and the pins in a pinball machine.
Intuition behind Quantile Loss
To understand the intuition behind quantile loss, let’s consider a simple example. Let’s say we want to predict the temperature for tomorrow. Our forecast predicts a range of 70-80 degrees with a 50% probability. This means that there is a 50% chance that the temperature will fall within this range. Now, if the actual temperature turns out to be 75 degrees, our quantile loss would be 0. This is because the actual value falls within the predicted range. However, if the actual temperature is 85 degrees, our quantile loss would be 10. This is because the actual value is outside the predicted range and the distance between the predicted 80th percentile and the actual value is 10.
Why is Quantile Loss Important?
Quantile loss is an important metric in probabilistic forecasting because it takes into account the uncertainty in the data. Traditional metrics like mean squared error or mean absolute error do not consider the uncertainty in the predictions. This can lead to misleading results, especially when dealing with highly volatile data. Quantile loss, on the other hand, provides a more accurate measure of forecast performance by considering the entire distribution of the data.
Conclusion
In conclusion, quantile loss, also known as pinball loss, is a metric used to evaluate the accuracy of probabilistic forecasts. It measures the difference between the
In summary, the quantile loss, also known as the pinball loss, is a metric that is used to evaluate the accuracy of probabilistic forecasts. It measures the difference between the predicted quantiles of a distribution and the actual observed values. By understanding the intuition behind this metric, we can better assess the performance of our forecasting models and make improvements to our predictions. With the use of quantile loss, we can ensure that our forecasts are reliable and provide valuable insights for decision making.
Discover the full story originally published on Towards Data Science.
Join us on this incredible generative AI journey and be a part of the revolution. Stay tuned for updates and insights on generative AI by following us on X or LinkedIn.